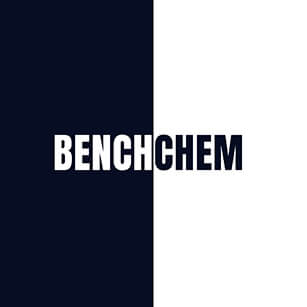
NIR-HS
- 点击 快速询问 获取最新报价。
- 提供有竞争力价格的高质量产品,您可以更专注于研究。
描述
NIR-HS is a highly selective and sensitive near-infrared fluorescent probe for imaging of hydrogen sulfide in living cells and in mice.
属性
分子式 |
C34H32IN3O6 |
---|---|
分子量 |
705.55 |
IUPAC 名称 |
(E)-2-(2-(6-(2,4-Dinitrophenoxy)-2,3-dihydro-1H-xanthen-4-yl)vinyl)-3,3-dimethyl-1-propyl-3H-indol-1-ium iodide |
InChI |
InChI=1S/C34H32N3O6.HI/c1-4-18-35-28-11-6-5-10-27(28)34(2,3)32(35)17-13-22-8-7-9-24-19-23-12-15-26(21-31(23)43-33(22)24)42-30-16-14-25(36(38)39)20-29(30)37(40)41;/h5-6,10-17,19-21H,4,7-9,18H2,1-3H3;1H/q+1;/p-1/b17-13+; |
InChI 键 |
OBQFEKRRPLICOT-IWSIBTJSSA-M |
SMILES |
CCC[N+]1=C(/C=C/C2=C3C(CCC2)=CC4=C(C=C(OC5=CC=C([N+]([O-])=O)C=C5[N+]([O-])=O)C=C4)O3)C(C)(C)C6=C1C=CC=C6.[I-] |
外观 |
Solid powder |
纯度 |
>98% (or refer to the Certificate of Analysis) |
保质期 |
>3 years if stored properly |
溶解度 |
Soluble in DMSO |
储存 |
Dry, dark and at 0 - 4 C for short term (days to weeks) or -20 C for long term (months to years). |
同义词 |
NIRHS; NIR HS; NIR-HS |
产品来源 |
United States |
Retrosynthesis Analysis
AI-Powered Synthesis Planning: Our tool employs the Template_relevance Pistachio, Template_relevance Bkms_metabolic, Template_relevance Pistachio_ringbreaker, Template_relevance Reaxys, Template_relevance Reaxys_biocatalysis model, leveraging a vast database of chemical reactions to predict feasible synthetic routes.
One-Step Synthesis Focus: Specifically designed for one-step synthesis, it provides concise and direct routes for your target compounds, streamlining the synthesis process.
Accurate Predictions: Utilizing the extensive PISTACHIO, BKMS_METABOLIC, PISTACHIO_RINGBREAKER, REAXYS, REAXYS_BIOCATALYSIS database, our tool offers high-accuracy predictions, reflecting the latest in chemical research and data.
Strategy Settings
Precursor scoring | Relevance Heuristic |
---|---|
Min. plausibility | 0.01 |
Model | Template_relevance |
Template Set | Pistachio/Bkms_metabolic/Pistachio_ringbreaker/Reaxys/Reaxys_biocatalysis |
Top-N result to add to graph | 6 |
Feasible Synthetic Routes
体外研究产品的免责声明和信息
请注意,BenchChem 上展示的所有文章和产品信息仅供信息参考。 BenchChem 上可购买的产品专为体外研究设计,这些研究在生物体外进行。体外研究,源自拉丁语 "in glass",涉及在受控实验室环境中使用细胞或组织进行的实验。重要的是要注意,这些产品没有被归类为药物或药品,他们没有得到 FDA 的批准,用于预防、治疗或治愈任何医疗状况、疾病或疾病。我们必须强调,将这些产品以任何形式引入人类或动物的身体都是法律严格禁止的。遵守这些指南对确保研究和实验的法律和道德标准的符合性至关重要。