The 2024 Nobel Prize in Chemistry is a victory for complex systems
The 2024 Nobel Prize in Chemistry has just been announced. David Baker, an American scientist, received half of the prize for his pioneering contributions to the field of protein structure design. The other half was jointly awarded to British scientist Demis Hassabis and American scientist John Jumper for their contributions to the field of protein structure prediction.
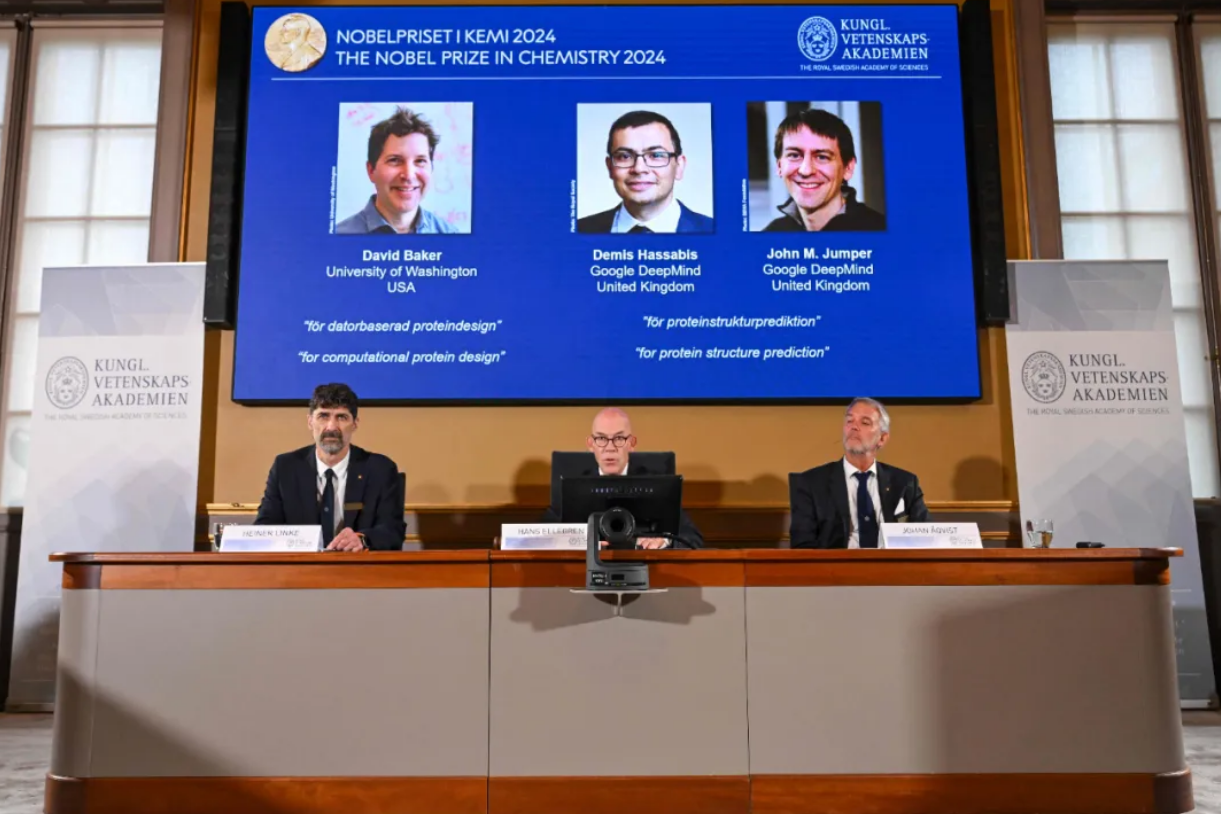
Both of these achievements are very new, but their goal is to solve a very old problem, which is how to predict the three-dimensional structure of a protein from its one-dimensional structure.
Scientists have long known that proteins are the most important chemical substances in living organisms, playing roles in everything from body structure to biochemical functions. Chemists have also long known that proteins are linear molecules made up of amino acids linked end-to-end, and they soon mastered the method of measuring protein amino acid sequences. However, the problem is that life is three-dimensional, and for proteins to function, they must first transform from a one-dimensional amino acid chain into a three-dimensional structure. The different functions of proteins are primarily due to their different three-dimensional structures.
To use an analogy, the same box of Lego bricks can be assembled into either a house or a car, and the value of the bricks to the player depends on the final result.
Common sense suggests that the three-dimensional structure of a protein should be determined by its one-dimensional structure, that is, its amino acid sequence. In the early years, some speculated that proteins folded into specific three-dimensional structures with the help of some external force, but in the 1960s, it was discovered that proteins could fold into specific structures in an appropriate solution without any external assistance, a process that takes less than a second. This is like saying that if you dismantle a Lego house, the blocks can instantly reassemble into the original house, looking exactly the same.
While this process seems magical, the principle is not complicated. It is known that amino acids are small molecules composed of atoms like carbon, hydrogen, oxygen, and nitrogen. These atoms are connected by chemical bonds, forming various groups that are hydrophilic, hydrophobic, or neutral. When protein molecules dissolve in water, the hydrophilic groups naturally expose themselves, while the hydrophobic groups tend to hide inside. In addition, some groups of amino acids are positively charged, while others are negatively charged, leading to attraction or repulsion reactions among them. If the position is not right, there will be a sense of tension within the protein, and only when each amino acid is located at the optimal position will the protein stabilize and reach its lowest energy state.
While the principle of protein folding is easy to understand, predicting the three-dimensional structure from the one-dimensional structure is extremely difficult due to the complexity and large number of calculations involved, as proteins are made up of hundreds or thousands of amino acids. Some of these amino acids form polypeptide chains, known as the primary structure of proteins; these polypeptide chains then fold into simple three-dimensional structures, such as helices or ladders, known in scientific terms as secondary structures; these simple secondary structures further combine into more complex three-dimensional conformations, known as the tertiary structure of proteins; finally, different proteins combine with some chemical elements to form even more complex protein complexes, which are the functional quaternary structures of proteins.
Scientists have found that they can only predict the secondary structure of a protein from its amino acid sequence, and further prediction becomes too complicated due to the vast computational requirements. The advent of X-ray crystallography partly solved this problem. In simple terms, scientists first purify the protein to be studied, then crystallize it under suitable conditions, and subsequently use X-ray diffraction to photograph it, from which the three-dimensional structure of the protein can be inferred. In fact, this technique can be applied to any large organic molecule, as evidenced by the elucidation of the DNA double helix structure.
The most proficient in this field is Chinese scientist Yan Ning, whose laboratory is an international leader in the prediction of protein three-dimensional structures, having published numerous papers. However, this technique requires first obtaining protein crystals, which is not an easy task, requiring significant time, effort, and cost. Moreover, many proteins are difficult to crystallize naturally, and many membrane proteins cannot be studied using this method, so this technical route can only solve part of the problem. Is there a way to directly predict the three-dimensional structure of a protein from its amino acid sequence? In theory, it is possible, but due to the vast amount of data and the complexity of the calculations, many scientists have invested in this field only to fail.
The first decisive breakthrough was achieved by David Baker, who won half of this year's Nobel Prize in Chemistry. He entered the field in 1993 and developed a series of software capable of predicting protein structures from amino acid sequences, named Rosetta. This software has maintained a leading position in the international protein structure prediction evaluation competition (CASP) for many years and was an early star in this field.
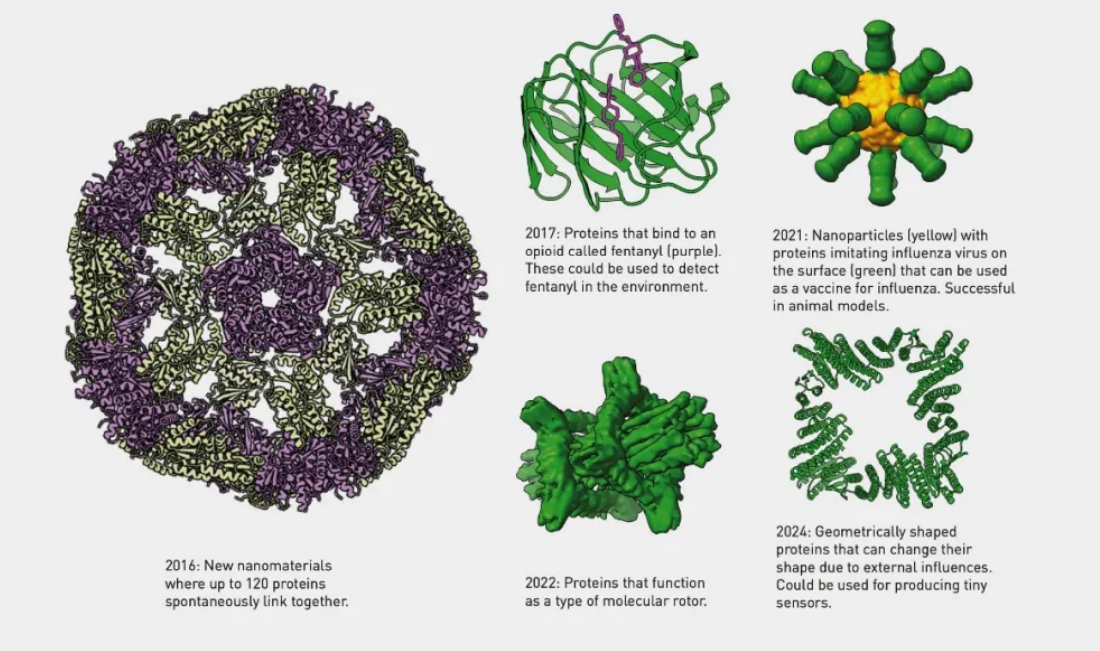
More commendable is that Baker did not attempt to monopolize this highly profitable technology. Instead, he established the Rosetta community, allowing anyone interested to download and contribute to the development of this software. This open-source spirit is extremely rare in today's money-driven world.
In the 2018 CASP competition, a mysterious contestant appeared: the renowned DeepMind team, famous for creating the Go-playing program AlphaGo. After their success, team leader Hassabis quickly shifted focus to the Mount Everest of the biological field—protein structure prediction. He hired a team of biologists and computer scientists to collaboratively tackle this problem. In 2018, they developed the first generation of AI-based protein structure prediction software, AlphaFold, which outperformed 97 other contestants in the 2018 CASP competition. In 2020, DeepMind developed AlphaFold2, which completely outclassed all previous prediction software, including Rosetta. The leader of the AlphaFold2 team, who shares the Nobel Prize with Hassabis, is Jumper. By 2021, AlphaFold2 was able to successfully predict 98.5% of human protein structures with accuracy comparable to experimental results. In May of this year, the DeepMind team released AlphaFold 3, predicting the 3D structures of nearly all life molecules, including proteins, DNA, and RNA, with unprecedented accuracy and understanding their interaction patterns.
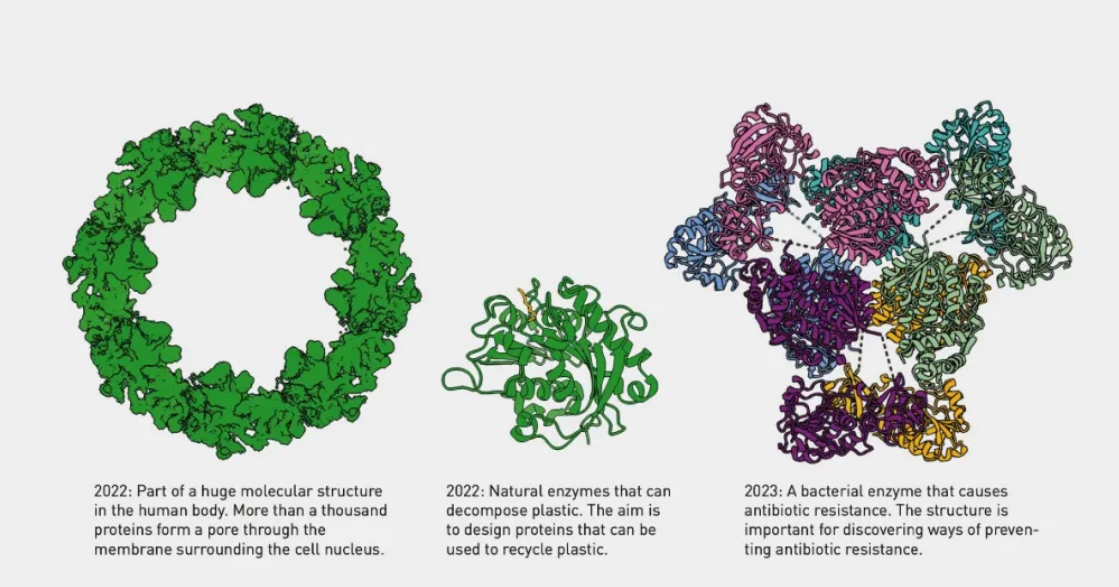
This achievement has immense potential in the field of new drug development. Scientists can now design new drugs on computers without laboriously searching for new drug candidates in nature. At this point, some readers may wonder if human scientists will no longer be needed in the future. At least for now, the answer is no. AI currently lacks the ability to generalize universal principles from complex phenomena or reach the level of the best human brains in this aspect. For example, many proteins change their properties when combined with a target molecule, a phenomenon known as allosteric regulation. About half of the proteins in the human body have the potential to be phosphorylated, but this is not a binary change; it is linear. Computers struggle to study this linear change. Furthermore, scientists have discovered many disordered proteins that do not have a definite 3D structure under natural conditions but have quite extensive functions. In fact, the structural flexibility of disordered proteins is essential for many biological functions, as proteins must continuously make linear adjustments to keep up with the ever-changing natural environment, a task currently difficult for computers to handle.
In short, this year's three Nobel Prizes in science are all related to complex systems. MicroRNA, which won the Nobel Prize in Physiology or Medicine, belongs to traditional classical genetics, but the gene regulation it represents is a highly complex system that is difficult to explain with simple causal chains. The neural networks that won the Nobel Prize in Physics are a computer simulation program of such complex systems, whose enormous potential we have already seen in the field of AI. The Chemistry Prize also reflects scientists' efforts to use computers to simulate the complex 3D structures of proteins, showing great potential in new drug development. Rather than saying that this year's Nobel Prizes are a victory for AI, it is more accurate to say it is a victory for complex systems. The paradigm of scientific research is evolving from deducing causal relationships to studying complex systems with unclear causal relationships. The absence of traditional physics in this year's awards also indicates that the scientific paradigm shift is already upon us.