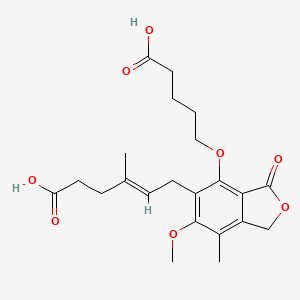
Mycophenolic Acid Carboxybutoxy Ether
Overview
Description
Mycophenolic Acid Carboxybutoxy Ether is an active form of mycophenolic acid. It has been shown to activate the immune system by increasing the production of lymphokines, including interleukin-2 and gamma-interferon . It has been shown to be effective in vivo in models for autoimmune diseases and cancer .
Synthesis Analysis
The biosynthesis of Mycophenolic Acid involves comparative incorporation experiments with (1′-14C)-orsellinic acid and (1′-14C)-4,6-dihydroxy-2,3-dimethylbenzoic acid, showing that the latter compound is a precursor of mycophenolic acid . The synthetic strategies for this potential lead compound was an evolution of various methods from generating the highly-substituted aromatic system via employing simple and commercially available materials to the catalytic methods employed .Molecular Structure Analysis
Mycophenolic Acid Carboxybutoxy Ether contains total 59 bond(s); 31 non-H bond(s), 10 multiple bond(s), 12 rotatable bond(s), 4 double bond(s), 6 aromatic bond(s), 1 five-membered ring(s), 1 six-membered ring(s), 1 nine-membered ring(s), 2 carboxylic acid(s) (aliphatic), 1 ester(s) (aromatic), 2 hydroxyl group(s), and 2 ether(s) (aromatic) .Physical And Chemical Properties Analysis
Mycophenolic Acid Carboxybutoxy Ether has a molecular weight of 420.5 g/mol . It has a total of 58 atoms; 28 Hydrogen atom(s), 22 Carbon atom(s), and 8 Oxygen atom(s) .Scientific Research Applications
Therapeutic Drug Monitoring (TDM) in Transplant Patients
MPAC is used as an internal standard in the quantification of MPA in plasma by High-Performance Liquid Chromatography-Tandem Mass Spectrometry (LC-MS/MS) . This is crucial for TDM in patients who have undergone solid organ transplants. Precise determination of systemic MPA concentration is significant due to the large inter-individual variability and narrow therapeutic range.
Immunopharmacology
MPA, the bioactive compound from which MPAC is derived, is metabolized in the liver, kidney, and intestines. It is involved in complex metabolic mechanisms, and approximately 97% of MPA and 82% of its glucuronide are bound to albumin in plasma . Only the free drug is biologically active, which underscores the importance of accurate measurement using standards like MPAC.
Anti-Influenza A Virus (IAV) Activity
Research has shown that derivatives of MPA, such as Mycophenolic Acid Methyl Ester (MAE), exhibit inhibitory effects against IAV strains . While not directly MPAC, this highlights the potential for MPAC to be used in similar antiviral research due to its structural similarity to MPA.
Activation of Cellular Pathways
MAE, a compound related to MPAC, has been observed to inhibit viral replication by activating the cellular Akt-mTOR-S6K pathway . This suggests that MPAC could be studied for its effects on cellular signaling pathways, which could have implications for both antiviral therapies and understanding cellular mechanisms.
Pharmacokinetics and Drug Metabolism
The metabolism of MPA involves conversion to various metabolites, with MPAC being used to understand these processes better. The study of MPAC can provide insights into the pharmacokinetics and drug metabolism of MPA, particularly in how it interacts with plasma proteins and undergoes enterohepatic recirculation .
Development of Novel Immunopharmacological Agents
Given the immunosuppressive nature of MPA, MPAC can be instrumental in the development of novel immunopharmacological agents. Its role as an internal standard in LC-MS/MS assays aids in the exploration of new therapeutic drugs that require precise dosing and monitoring .
Mechanism of Action
Target of Action
Mycophenolic Acid Carboxybutoxy Ether (MPAC) is an active form of Mycophenolic Acid (MPA), a potent immunosuppressant . The primary target of MPA is the enzyme inosine monophosphate dehydrogenase (IMPDH), which plays a crucial role in the de novo synthesis of guanosine nucleotides within lymphocytes . By inhibiting IMPDH, MPA interferes with the proliferation of T- and B-lymphocytes, which are key players in the immune response .
Mode of Action
MPA is a selective, noncompetitive, and reversible inhibitor of IMPDH . It blocks the conversion of inosine-5-phosphate and xanthine-5-phosphate to guanosine-5-phosphate . This inhibition disrupts the de novo pathway of guanosine nucleotide biosynthesis, leading to a decrease in DNA and RNA synthesis and, consequently, a reduction in lymphocyte proliferation .
Biochemical Pathways
MPA is metabolized in the liver, kidney, and intestines by the Uridine 50-diphospho (UDP)—glucuronosyltransferase enzyme into 7-O-mycophenolic acid glucuronide (MPAG) and mycophenolic acid acyl-glucuronide (AcMPAG) . MPAG has minimal pharmacological activity, while AcMPAG has been observed to induce pro-inflammatory and toxic effects .
Pharmacokinetics
MPA is predominantly metabolized by glucuronidation to form an inactive metabolite, mycophenolic acid glucuronide (MPAG), as well as a minor acyl glucuronide metabolite . The extent of metabolism varies among individuals, and the MPAG formed can be hydrolyzed to MPA and can displace MPA from serum albumin, creating a potential need to monitor both MPA and MPAG .
Result of Action
The inhibition of IMPDH by MPA leads to potent cytostatic effects on T- and B-lymphocytes . MPA also suppresses antibody formation by B-lymphocytes and prevents the glycosylation of lymphocyte and monocyte glycoproteins involved in intercellular adhesion to endothelial cells . This results in a strong immunosuppressive effect, making MPA useful in preventing organ transplant rejections .
Action Environment
The action of MPA can be influenced by various environmental factors. For instance, the conversion of MPAG back into MPA can occur through the enterohepatic recirculation due to deglucuronidation by enteric bacteria . This complex metabolic mechanism can lead to variable therapeutic dosing between patients . Furthermore, approximately 97% of MPA and 82% of MPAG are bound to albumin in plasma , indicating that changes in plasma protein levels can affect the drug’s bioavailability.
Safety and Hazards
properties
IUPAC Name |
(E)-6-[4-(4-carboxybutoxy)-6-methoxy-7-methyl-3-oxo-1H-2-benzofuran-5-yl]-4-methylhex-4-enoic acid | |
---|---|---|
Source | PubChem | |
URL | https://pubchem.ncbi.nlm.nih.gov | |
Description | Data deposited in or computed by PubChem | |
InChI |
InChI=1S/C22H28O8/c1-13(8-10-18(25)26)7-9-15-20(28-3)14(2)16-12-30-22(27)19(16)21(15)29-11-5-4-6-17(23)24/h7H,4-6,8-12H2,1-3H3,(H,23,24)(H,25,26)/b13-7+ | |
Source | PubChem | |
URL | https://pubchem.ncbi.nlm.nih.gov | |
Description | Data deposited in or computed by PubChem | |
InChI Key |
PFECETFRFNZFKJ-NTUHNPAUSA-N | |
Source | PubChem | |
URL | https://pubchem.ncbi.nlm.nih.gov | |
Description | Data deposited in or computed by PubChem | |
Canonical SMILES |
CC1=C2COC(=O)C2=C(C(=C1OC)CC=C(C)CCC(=O)O)OCCCCC(=O)O | |
Source | PubChem | |
URL | https://pubchem.ncbi.nlm.nih.gov | |
Description | Data deposited in or computed by PubChem | |
Isomeric SMILES |
CC1=C2COC(=O)C2=C(C(=C1OC)C/C=C(\C)/CCC(=O)O)OCCCCC(=O)O | |
Source | PubChem | |
URL | https://pubchem.ncbi.nlm.nih.gov | |
Description | Data deposited in or computed by PubChem | |
Molecular Formula |
C22H28O8 | |
Source | PubChem | |
URL | https://pubchem.ncbi.nlm.nih.gov | |
Description | Data deposited in or computed by PubChem | |
DSSTOX Substance ID |
DTXSID10858155 | |
Record name | (4E)-6-[4-(4-Carboxybutoxy)-6-methoxy-7-methyl-3-oxo-1,3-dihydro-2-benzofuran-5-yl]-4-methylhex-4-enoic acid | |
Source | EPA DSSTox | |
URL | https://comptox.epa.gov/dashboard/DTXSID10858155 | |
Description | DSSTox provides a high quality public chemistry resource for supporting improved predictive toxicology. | |
Molecular Weight |
420.5 g/mol | |
Source | PubChem | |
URL | https://pubchem.ncbi.nlm.nih.gov | |
Description | Data deposited in or computed by PubChem | |
Product Name |
Mycophenolic Acid Carboxybutoxy Ether | |
CAS RN |
931407-27-1 | |
Record name | (4E)-6-[4-(4-Carboxybutoxy)-6-methoxy-7-methyl-3-oxo-1,3-dihydro-2-benzofuran-5-yl]-4-methylhex-4-enoic acid | |
Source | EPA DSSTox | |
URL | https://comptox.epa.gov/dashboard/DTXSID10858155 | |
Description | DSSTox provides a high quality public chemistry resource for supporting improved predictive toxicology. | |
Q & A
Q1: Why is Mycophenolic Acid Carboxybutoxy Ether used as an internal standard in the analysis of Mycophenolic Acid?
A1: Mycophenolic Acid Carboxybutoxy Ether is chosen as a suitable internal standard for this analysis due to its structural similarity to Mycophenolic Acid and its derivatives []. This similarity in structure often translates to similar chromatographic behavior, meaning it will elute close to the analytes of interest (Mycophenolic Acid, Mycophenolic Acid phenol glucoside, Mycophenolic Acid phenol glucuronide). By comparing the peak area ratios of the analytes to the internal standard, researchers can achieve more accurate and reliable quantification, even with potential variations during sample preparation or analysis.
Q2: What are the advantages of the presented UHPLC-UV method for analyzing Mycophenolic Acid and its metabolites in canine and feline plasma?
A2: The research highlights several advantages of the developed Ultra High Performance Liquid Chromatography - Ultraviolet (UHPLC-UV) method for this specific application []:
Disclaimer and Information on In-Vitro Research Products
Please be aware that all articles and product information presented on BenchChem are intended solely for informational purposes. The products available for purchase on BenchChem are specifically designed for in-vitro studies, which are conducted outside of living organisms. In-vitro studies, derived from the Latin term "in glass," involve experiments performed in controlled laboratory settings using cells or tissues. It is important to note that these products are not categorized as medicines or drugs, and they have not received approval from the FDA for the prevention, treatment, or cure of any medical condition, ailment, or disease. We must emphasize that any form of bodily introduction of these products into humans or animals is strictly prohibited by law. It is essential to adhere to these guidelines to ensure compliance with legal and ethical standards in research and experimentation.