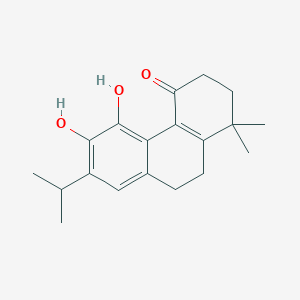
Sageone
Overview
Description
Preparation Methods
Synthetic Routes and Reaction Conditions: Sageone can be isolated from the n-hexane fraction of the aqueous methanol extract of Salvia miltiorrhiza through activity-guided fractionation and repeated chromatographic separation over silica gel, RP C18, and Sephadex LH-20 . The structures of the isolated compounds are determined using nuclear magnetic resonance spectroscopy, mass spectroscopy, and infrared spectroscopy .
Industrial Production Methods: Currently, there is limited information on the large-scale industrial production of this compound. The extraction and purification processes used in research settings may need to be optimized for industrial applications.
Chemical Reactions Analysis
Types of Reactions: Sageone undergoes various chemical reactions, including oxidation, reduction, and substitution reactions. These reactions are essential for modifying the compound’s structure and enhancing its biological activity.
Common Reagents and Conditions:
Oxidation: Common oxidizing agents such as potassium permanganate or chromium trioxide can be used to oxidize this compound.
Reduction: Reducing agents like sodium borohydride or lithium aluminum hydride are employed to reduce this compound.
Substitution: Halogenation and other substitution reactions can be carried out using reagents like halogens or nucleophiles under appropriate conditions.
Major Products: The major products formed from these reactions depend on the specific reagents and conditions used. For example, oxidation may yield hydroxylated derivatives, while reduction can produce deoxygenated compounds.
Scientific Research Applications
Sageone has a wide range of scientific research applications, including:
Industry: While its industrial applications are still under investigation, this compound’s unique properties make it a candidate for developing new pharmaceuticals and therapeutic agents.
Mechanism of Action
Sageone exerts its effects through multiple molecular targets and pathways. It has been shown to reduce the expression of Akt, a protein kinase involved in cell survival and proliferation . This reduction leads to increased apoptosis in cancer cells, particularly when combined with cisplatin . This compound also induces the cleavage of caspase-3, -9, and poly (ADP-ribose) polymerase, further promoting apoptosis .
Comparison with Similar Compounds
Sageone is unique among diterpenoids due to its specific molecular structure and biological activity. Similar compounds include:
Barbatusol: An icetexane diterpenoid with cytotoxic properties.
Verbenone: A monoterpene with antiviral and cytotoxic activities.
Compared to these compounds, this compound exhibits a more potent synergistic effect with cisplatin, making it a promising candidate for combination therapy in cancer treatment .
Biological Activity
Sageone, chemically known as 5,6-dihydroxy-1,1-dimethyl-7-(propan-2-yl)-1,2,3,4,9,10-hexahydrophenanthren-4-one (CAS Number: 142546-15-4), is a diterpenoid compound derived primarily from Salvia officinalis (common sage) and related species. Its unique structure and biological properties have garnered significant attention in pharmacological research, particularly for its potential anticancer effects.
Chemical Structure and Properties
This compound's chemical formula is . It is characterized by a complex aromatic structure formed from four isoprene units. This structural uniqueness contributes to its diverse biological activities.
Biological Activities
This compound exhibits a range of biological activities that are primarily focused on its cytotoxic effects against cancer cells and its potential as an antioxidant. Key findings include:
- Cytotoxicity : this compound has demonstrated significant cytotoxic effects against various cancer cell lines. Notably, it has shown an IC50 value of against SNU-1 human gastric cancer cells, indicating its potential as an antitumor agent.
- Synergistic Effects : Research indicates that this compound can enhance the efficacy of conventional chemotherapeutic agents such as cisplatin. This synergy is believed to occur through the modulation of signaling pathways, particularly the Akt pathway, which plays a crucial role in cell survival and proliferation.
- Antioxidant Properties : The compound also exhibits antioxidant activity, which may help mitigate oxidative stress in cells. This property is particularly relevant in the context of inflammation and other oxidative stress-related conditions.
This compound's biological activity is mediated through multiple molecular targets:
- Cell Signaling Modulation : It has been shown to reduce the expression of Akt in cancer cells, which may help overcome drug resistance mechanisms commonly observed in cancer therapies.
- Lipid Peroxidation : The compound participates in lipid metabolism and can induce lipid peroxidation, leading to oxidative damage in cells. This reaction has implications for cellular health and disease mechanisms.
Comparative Analysis with Similar Compounds
To better understand this compound's unique properties, it can be compared with other diterpenoids found in Salvia species:
Compound Name | Chemical Structure | Unique Features |
---|---|---|
Rosmarinic Acid | Known for anti-inflammatory and antioxidant effects | |
Carnosic Acid | Exhibits neuroprotective properties | |
Abietic Acid | Commonly used in resins with antimicrobial effects |
Uniqueness of this compound : Unlike these compounds, this compound's specific interactions with cancer cell signaling pathways position it as a promising candidate for therapeutic development in oncology.
Case Studies and Research Findings
Several studies have investigated the biological activities of this compound:
- Cytotoxicity Studies : A study conducted on SNU-1 cells revealed that this compound not only inhibited cell growth but also induced apoptosis through the activation of specific apoptotic pathways.
- Combination Therapy Research : In vitro experiments demonstrated that combining this compound with cisplatin significantly increased cytotoxicity compared to cisplatin alone. This suggests that this compound could be integrated into combination therapy regimens for enhanced efficacy against resistant cancer types.
- Antioxidant Activity Assessment : Various assays have confirmed this compound's ability to scavenge free radicals, contributing to its potential use in preventing oxidative stress-related diseases.
Properties
IUPAC Name |
5,6-dihydroxy-1,1-dimethyl-7-propan-2-yl-2,3,9,10-tetrahydrophenanthren-4-one | |
---|---|---|
Source | PubChem | |
URL | https://pubchem.ncbi.nlm.nih.gov | |
Description | Data deposited in or computed by PubChem | |
InChI |
InChI=1S/C19H24O3/c1-10(2)12-9-11-5-6-13-16(15(11)18(22)17(12)21)14(20)7-8-19(13,3)4/h9-10,21-22H,5-8H2,1-4H3 | |
Source | PubChem | |
URL | https://pubchem.ncbi.nlm.nih.gov | |
Description | Data deposited in or computed by PubChem | |
InChI Key |
NPQAMUFQEFLLCY-UHFFFAOYSA-N | |
Source | PubChem | |
URL | https://pubchem.ncbi.nlm.nih.gov | |
Description | Data deposited in or computed by PubChem | |
Canonical SMILES |
CC(C)C1=C(C(=C2C(=C1)CCC3=C2C(=O)CCC3(C)C)O)O | |
Source | PubChem | |
URL | https://pubchem.ncbi.nlm.nih.gov | |
Description | Data deposited in or computed by PubChem | |
Molecular Formula |
C19H24O3 | |
Source | PubChem | |
URL | https://pubchem.ncbi.nlm.nih.gov | |
Description | Data deposited in or computed by PubChem | |
Molecular Weight |
300.4 g/mol | |
Source | PubChem | |
URL | https://pubchem.ncbi.nlm.nih.gov | |
Description | Data deposited in or computed by PubChem | |
CAS No. |
142546-15-4 | |
Record name | Sageone | |
Source | CAS Common Chemistry | |
URL | https://commonchemistry.cas.org/detail?cas_rn=142546-15-4 | |
Description | CAS Common Chemistry is an open community resource for accessing chemical information. Nearly 500,000 chemical substances from CAS REGISTRY cover areas of community interest, including common and frequently regulated chemicals, and those relevant to high school and undergraduate chemistry classes. This chemical information, curated by our expert scientists, is provided in alignment with our mission as a division of the American Chemical Society. | |
Explanation | The data from CAS Common Chemistry is provided under a CC-BY-NC 4.0 license, unless otherwise stated. | |
Record name | Sageone | |
Source | Human Metabolome Database (HMDB) | |
URL | http://www.hmdb.ca/metabolites/HMDB0038684 | |
Description | The Human Metabolome Database (HMDB) is a freely available electronic database containing detailed information about small molecule metabolites found in the human body. | |
Explanation | HMDB is offered to the public as a freely available resource. Use and re-distribution of the data, in whole or in part, for commercial purposes requires explicit permission of the authors and explicit acknowledgment of the source material (HMDB) and the original publication (see the HMDB citing page). We ask that users who download significant portions of the database cite the HMDB paper in any resulting publications. | |
Retrosynthesis Analysis
AI-Powered Synthesis Planning: Our tool employs the Template_relevance Pistachio, Template_relevance Bkms_metabolic, Template_relevance Pistachio_ringbreaker, Template_relevance Reaxys, Template_relevance Reaxys_biocatalysis model, leveraging a vast database of chemical reactions to predict feasible synthetic routes.
One-Step Synthesis Focus: Specifically designed for one-step synthesis, it provides concise and direct routes for your target compounds, streamlining the synthesis process.
Accurate Predictions: Utilizing the extensive PISTACHIO, BKMS_METABOLIC, PISTACHIO_RINGBREAKER, REAXYS, REAXYS_BIOCATALYSIS database, our tool offers high-accuracy predictions, reflecting the latest in chemical research and data.
Strategy Settings
Precursor scoring | Relevance Heuristic |
---|---|
Min. plausibility | 0.01 |
Model | Template_relevance |
Template Set | Pistachio/Bkms_metabolic/Pistachio_ringbreaker/Reaxys/Reaxys_biocatalysis |
Top-N result to add to graph | 6 |
Feasible Synthetic Routes
Q1: What are the known biological activities of Sageone?
A1: this compound has shown promising activity in interacting with both cannabinoid and opioid receptors. Research indicates that it can displace both CB1 [, ] and CB2 [] receptors, suggesting potential therapeutic applications related to these systems. Additionally, this compound exhibits moderate activity towards the mu opioid receptor [], further expanding its potential therapeutic scope.
Q2: From what natural sources can this compound be isolated?
A3: this compound has been successfully isolated from Salvia apiana (white sage) [, ] and Rosmarinus officinalis (rosemary) []. These plants are recognized for their diverse phytochemical profiles and traditional uses in various cultures.
Q3: Are there any studies investigating the synergistic effects of this compound with other compounds?
A4: Yes, research indicates that this compound synergizes with cisplatin, a chemotherapy drug, in SNU-1 human gastric cancer cells [, ]. This synergistic effect highlights the potential of this compound to enhance the efficacy of existing cancer treatments.
Q4: Has this compound been explored as a potential antiviral agent?
A5: Yes, computational docking studies suggest that this compound can directly bind to both the human ACE2 receptor and the viral main protease, key targets in SARS-CoV-2 infection [, ]. These findings point to the potential of this compound as an antiviral agent, although further experimental validation is needed.
Q5: Have any studies examined the potential toxicity of this compound?
A6: The provided abstracts don't offer specific data on the toxicity of this compound. While some studies mention potential cytotoxic effects of other compounds isolated alongside this compound [], further research is necessary to establish the safety profile of this compound itself.
Q6: What analytical techniques are commonly employed to characterize and quantify this compound?
A7: Researchers commonly utilize a combination of spectroscopic and chromatographic techniques to characterize and quantify this compound. These techniques include Nuclear Magnetic Resonance (NMR) spectroscopy [, ], Mass Spectrometry (MS) [, ], and various chromatographic methods such as column chromatography [, ].
Disclaimer and Information on In-Vitro Research Products
Please be aware that all articles and product information presented on BenchChem are intended solely for informational purposes. The products available for purchase on BenchChem are specifically designed for in-vitro studies, which are conducted outside of living organisms. In-vitro studies, derived from the Latin term "in glass," involve experiments performed in controlled laboratory settings using cells or tissues. It is important to note that these products are not categorized as medicines or drugs, and they have not received approval from the FDA for the prevention, treatment, or cure of any medical condition, ailment, or disease. We must emphasize that any form of bodily introduction of these products into humans or animals is strictly prohibited by law. It is essential to adhere to these guidelines to ensure compliance with legal and ethical standards in research and experimentation.