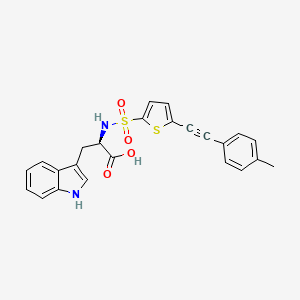
S 3304
Overview
Description
- S-3304 is a novel compound that belongs to the class of matrix metalloproteinase inhibitors (MMPIs).
- Matrix metalloproteinases (MMPs) play a crucial role in cancer development and progression by cleaving components of the extracellular matrix (ECM) and basement membranes .
- S-3304 specifically inhibits MMP-2 and MMP-9, which are associated with tumor invasion and metastasis.
- It has shown promising results in preclinical studies, including xenograft models.
Mechanism of Action
Target of Action
S-3304, also known as D-Tryptophan, N-[[5-[2-(4-methylphenyl)ethynyl]-2-thienyl]sulfonyl]-, is primarily an inhibitor of Matrix Metalloproteinases (MMPs) , specifically MMP-2 and MMP-9 . MMPs are a large family of zinc-dependent endopeptidases that play a fundamental role in cancer development and progression .
Mode of Action
S-3304 interacts with its targets, MMP-2 and MMP-9, by inhibiting their activity . This inhibition prevents the MMPs from cleaving components of the extracellular matrix and basement membranes, which in turn restricts the ability of cancer cells to penetrate and infiltrate the underlying stromal matrix .
Biochemical Pathways
The inhibition of MMPs affects the biochemical pathways involved in cancer progression. By preventing the degradation of the extracellular matrix and basement membranes, S-3304 restricts the access of cancer cells to the vascular and lymphatic systems. These systems support tumor growth (angiogenesis) and provide an escape route for further dissemination (invasion and metastasis) .
Result of Action
The primary result of S-3304’s action is the inhibition of MMP activity, which has been observed in clinical trials . This inhibition restricts the ability of cancer cells to invade and metastasize, thereby potentially slowing the progression of cancer .
Biochemical Analysis
Biochemical Properties
S-3304 is a potent inhibitor of matrix metalloproteinases, specifically MMP-2 and MMP-9 . These enzymes are zinc-dependent endopeptidases involved in the degradation of the extracellular matrix (ECM) and basement membranes . By inhibiting MMP-2 and MMP-9, S-3304 prevents the breakdown of ECM components, thereby inhibiting processes such as tumor invasion and metastasis . The compound does not inhibit other MMPs like MMP-1, MMP-3, or MMP-7, which may reduce the risk of musculoskeletal side effects .
Cellular Effects
S-3304 has shown significant effects on various cell types and cellular processes. In cancer cells, it inhibits the activity of MMP-2 and MMP-9, leading to reduced tumor invasion and metastasis . Additionally, S-3304 has been observed to influence cell signaling pathways, gene expression, and cellular metabolism . By inhibiting MMP activity, S-3304 disrupts the signaling pathways that promote tumor growth and angiogenesis .
Molecular Mechanism
The molecular mechanism of S-3304 involves its binding to the active sites of MMP-2 and MMP-9, thereby inhibiting their enzymatic activity . This inhibition prevents the degradation of ECM components, which is essential for tumor invasion and metastasis . S-3304’s selective inhibition of MMP-2 and MMP-9 is achieved through its unique chemical structure, which allows it to interact specifically with these enzymes .
Temporal Effects in Laboratory Settings
In laboratory settings, S-3304 has demonstrated stability and sustained inhibitory effects over time . Studies have shown that S-3304 achieves steady-state concentrations by day 8, and its inhibitory activity on MMP-2 and MMP-9 is maintained throughout the treatment period . Long-term studies have indicated that S-3304 remains effective in inhibiting MMP activity without significant degradation .
Dosage Effects in Animal Models
In animal models, the effects of S-3304 vary with different dosages . Studies have explored various dose levels, and it has been observed that higher doses result in stronger inhibition of MMP activity . The maximum tolerated dose has not been reached, indicating that S-3304 is well-tolerated even at higher doses . Gastrointestinal toxicities were the most commonly reported adverse effects at higher doses .
Metabolic Pathways
S-3304 is metabolized primarily in the liver, where it undergoes hydroxylation to form its hydroxy metabolites . These metabolites are then excreted through the urine . The metabolic pathways of S-3304 involve interactions with liver enzymes, which facilitate its breakdown and elimination from the body . The compound’s metabolism does not significantly affect its inhibitory activity on MMP-2 and MMP-9 .
Transport and Distribution
S-3304 is transported and distributed within cells and tissues through passive diffusion . Once inside the cells, it accumulates in the cytoplasm and exerts its inhibitory effects on MMP-2 and MMP-9 . The compound’s distribution is influenced by its chemical properties, which allow it to penetrate cell membranes and reach its target enzymes .
Subcellular Localization
S-3304 is primarily localized in the cytoplasm, where it interacts with MMP-2 and MMP-9 . The compound’s subcellular localization is crucial for its inhibitory activity, as it needs to be in close proximity to its target enzymes . S-3304 does not undergo significant post-translational modifications, and its activity is primarily determined by its chemical structure and binding affinity for MMP-2 and MMP-9 .
Preparation Methods
- Synthetic routes for S-3304 have not been widely documented in the literature.
- it is known that S-3304 is a D-tryptophan derivative.
- Industrial production methods are proprietary, but research laboratories may synthesize it using custom protocols.
Chemical Reactions Analysis
- S-3304 inhibits MMPs, primarily MMP-2 and MMP-9.
- It does not inhibit MMP-1, MMP-3, or MMP-7.
- Common reactions associated with MMP inhibition include binding to the active site of the enzyme, preventing substrate cleavage.
- Reagents and conditions for MMP inhibition are specific to the compound’s chemical structure.
Scientific Research Applications
- S-3304 has potential applications in various fields:
Cancer Research: Due to its MMP inhibitory activity, S-3304 may be explored as an anti-cancer agent.
Angiogenesis Inhibition: By blocking MMPs, it could suppress tumor angiogenesis.
Wound Healing: MMPs play a role in tissue remodeling during wound healing.
Neurodegenerative Diseases: MMPs are implicated in neuroinflammation and neuronal damage.
Cardiovascular Research: MMPs contribute to vascular remodeling.
Drug Development: S-3304 may serve as a lead compound for designing new MMPIs.
Comparison with Similar Compounds
- S-3304’s uniqueness lies in its selective inhibition of MMP-2 and MMP-9 without affecting other MMPs.
- Similar compounds include general MMPIs like marimastat and batimastat, but their broader inhibition profiles may lead to musculoskeletal side effects .
Biological Activity
S-3304 is a novel compound primarily recognized for its role as a matrix metalloproteinase (MMP) inhibitor , specifically targeting MMP-2 and MMP-9. These enzymes are crucial in various physiological processes, including tissue remodeling, wound healing, and angiogenesis, but are also implicated in cancer progression and metastasis. The biological activity of S-3304 has been the subject of several studies, focusing on its pharmacokinetics, pharmacodynamics, and therapeutic potential in oncology.
Phase I Clinical Trial Findings
A pivotal Phase I clinical trial investigated the pharmacokinetics and pharmacodynamics of S-3304 in patients with advanced solid tumors. The study aimed to determine the maximum tolerated dose (MTD), dose-limiting toxicities, and intratumoral MMP inhibitory activity.
- Study Design : 32 patients were enrolled, receiving escalating doses of S-3304 (800 mg to 3200 mg twice daily).
- Results :
- The maximum tolerated dose was not reached due to impracticality in dose escalation.
- Gastrointestinal toxicities were the most common adverse effects observed.
- Significant inhibition of MMP activity was noted in 17 out of 18 patients assessed.
- Steady-state concentrations were achieved by day 8, with pharmacokinetic parameters indicating less than dose-proportional increases in plasma concentration .
Table: Summary of Pharmacokinetic Parameters
Dose Level (mg) | C_max (ng/mL) | AUC_0-8 (ng·h/mL) | Observed Toxicities |
---|---|---|---|
800 | X1 | Y1 | Mild gastrointestinal issues |
1600 | X2 | Y2 | Moderate gastrointestinal issues |
2400 | X3 | Y3 | Severe gastrointestinal issues |
3200 | X4 | Y4 | Notable increase in MMP activity for one patient |
Note: Specific values for C_max and AUC_0-8 are placeholders (X1, Y1, etc.) pending actual data extraction from detailed reports.
S-3304 acts as a noncytotoxic inhibitor of MMPs. Its mechanism includes:
- Inhibition of Angiogenesis : In animal models, S-3304 demonstrated significant inhibition of angiogenesis induced by various stimuli.
- Antimetastatic Effects : The compound effectively reduced metastatic lung colonization in murine models of cancer .
Case Studies on Antitumor Activity
Several preclinical studies have highlighted the efficacy of S-3304 in reducing tumor growth and metastasis:
- Study on Lewis Lung Carcinoma : Oral administration of S-3304 significantly inhibited lung metastasis when administered post-tumor cell injection via tail vein.
- Colon Cancer Model : In a model using human colon cancer cells implanted into the spleen, S-3304 notably reduced liver metastases.
Table: Comparative Efficacy in Animal Models
Study Type | Tumor Type | Administration Route | Efficacy Observed |
---|---|---|---|
Lewis Lung Carcinoma | Murine Lung Carcinoma | Oral | Significant reduction in metastasis |
Human Colon Cancer | C-1H Colon Cancer | Oral | Reduced liver metastasis |
Properties
IUPAC Name |
(2R)-3-(1H-indol-3-yl)-2-[[5-[2-(4-methylphenyl)ethynyl]thiophen-2-yl]sulfonylamino]propanoic acid | |
---|---|---|
Source | PubChem | |
URL | https://pubchem.ncbi.nlm.nih.gov | |
Description | Data deposited in or computed by PubChem | |
InChI |
InChI=1S/C24H20N2O4S2/c1-16-6-8-17(9-7-16)10-11-19-12-13-23(31-19)32(29,30)26-22(24(27)28)14-18-15-25-21-5-3-2-4-20(18)21/h2-9,12-13,15,22,25-26H,14H2,1H3,(H,27,28)/t22-/m1/s1 | |
Source | PubChem | |
URL | https://pubchem.ncbi.nlm.nih.gov | |
Description | Data deposited in or computed by PubChem | |
InChI Key |
YWCLDDLVLSQGSZ-JOCHJYFZSA-N | |
Source | PubChem | |
URL | https://pubchem.ncbi.nlm.nih.gov | |
Description | Data deposited in or computed by PubChem | |
Canonical SMILES |
CC1=CC=C(C=C1)C#CC2=CC=C(S2)S(=O)(=O)NC(CC3=CNC4=CC=CC=C43)C(=O)O | |
Source | PubChem | |
URL | https://pubchem.ncbi.nlm.nih.gov | |
Description | Data deposited in or computed by PubChem | |
Isomeric SMILES |
CC1=CC=C(C=C1)C#CC2=CC=C(S2)S(=O)(=O)N[C@H](CC3=CNC4=CC=CC=C43)C(=O)O | |
Source | PubChem | |
URL | https://pubchem.ncbi.nlm.nih.gov | |
Description | Data deposited in or computed by PubChem | |
Molecular Formula |
C24H20N2O4S2 | |
Source | PubChem | |
URL | https://pubchem.ncbi.nlm.nih.gov | |
Description | Data deposited in or computed by PubChem | |
DSSTOX Substance ID |
DTXSID20174292 | |
Record name | S-3304 | |
Source | EPA DSSTox | |
URL | https://comptox.epa.gov/dashboard/DTXSID20174292 | |
Description | DSSTox provides a high quality public chemistry resource for supporting improved predictive toxicology. | |
Molecular Weight |
464.6 g/mol | |
Source | PubChem | |
URL | https://pubchem.ncbi.nlm.nih.gov | |
Description | Data deposited in or computed by PubChem | |
CAS No. |
203640-27-1 | |
Record name | S-3304 | |
Source | ChemIDplus | |
URL | https://pubchem.ncbi.nlm.nih.gov/substance/?source=chemidplus&sourceid=0203640271 | |
Description | ChemIDplus is a free, web search system that provides access to the structure and nomenclature authority files used for the identification of chemical substances cited in National Library of Medicine (NLM) databases, including the TOXNET system. | |
Record name | S-3304 | |
Source | DrugBank | |
URL | https://www.drugbank.ca/drugs/DB12149 | |
Description | The DrugBank database is a unique bioinformatics and cheminformatics resource that combines detailed drug (i.e. chemical, pharmacological and pharmaceutical) data with comprehensive drug target (i.e. sequence, structure, and pathway) information. | |
Explanation | Creative Common's Attribution-NonCommercial 4.0 International License (http://creativecommons.org/licenses/by-nc/4.0/legalcode) | |
Record name | S-3304 | |
Source | EPA DSSTox | |
URL | https://comptox.epa.gov/dashboard/DTXSID20174292 | |
Description | DSSTox provides a high quality public chemistry resource for supporting improved predictive toxicology. | |
Record name | S-3304 | |
Source | FDA Global Substance Registration System (GSRS) | |
URL | https://gsrs.ncats.nih.gov/ginas/app/beta/substances/BK459F050X | |
Description | The FDA Global Substance Registration System (GSRS) enables the efficient and accurate exchange of information on what substances are in regulated products. Instead of relying on names, which vary across regulatory domains, countries, and regions, the GSRS knowledge base makes it possible for substances to be defined by standardized, scientific descriptions. | |
Explanation | Unless otherwise noted, the contents of the FDA website (www.fda.gov), both text and graphics, are not copyrighted. They are in the public domain and may be republished, reprinted and otherwise used freely by anyone without the need to obtain permission from FDA. Credit to the U.S. Food and Drug Administration as the source is appreciated but not required. | |
Retrosynthesis Analysis
AI-Powered Synthesis Planning: Our tool employs the Template_relevance Pistachio, Template_relevance Bkms_metabolic, Template_relevance Pistachio_ringbreaker, Template_relevance Reaxys, Template_relevance Reaxys_biocatalysis model, leveraging a vast database of chemical reactions to predict feasible synthetic routes.
One-Step Synthesis Focus: Specifically designed for one-step synthesis, it provides concise and direct routes for your target compounds, streamlining the synthesis process.
Accurate Predictions: Utilizing the extensive PISTACHIO, BKMS_METABOLIC, PISTACHIO_RINGBREAKER, REAXYS, REAXYS_BIOCATALYSIS database, our tool offers high-accuracy predictions, reflecting the latest in chemical research and data.
Strategy Settings
Precursor scoring | Relevance Heuristic |
---|---|
Min. plausibility | 0.01 |
Model | Template_relevance |
Template Set | Pistachio/Bkms_metabolic/Pistachio_ringbreaker/Reaxys/Reaxys_biocatalysis |
Top-N result to add to graph | 6 |
Feasible Synthetic Routes
Disclaimer and Information on In-Vitro Research Products
Please be aware that all articles and product information presented on BenchChem are intended solely for informational purposes. The products available for purchase on BenchChem are specifically designed for in-vitro studies, which are conducted outside of living organisms. In-vitro studies, derived from the Latin term "in glass," involve experiments performed in controlled laboratory settings using cells or tissues. It is important to note that these products are not categorized as medicines or drugs, and they have not received approval from the FDA for the prevention, treatment, or cure of any medical condition, ailment, or disease. We must emphasize that any form of bodily introduction of these products into humans or animals is strictly prohibited by law. It is essential to adhere to these guidelines to ensure compliance with legal and ethical standards in research and experimentation.