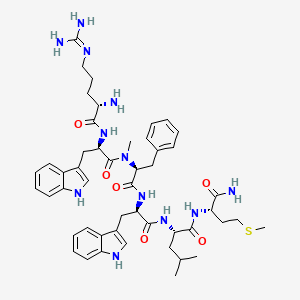
Antagonist G
Overview
Description
- Additionally, this compound weakly antagonizes gastrin-releasing peptide (GRP) and bradykinin.
- Notably, it can induce the transcription of AG-1, making cancer cells more sensitive to chemotherapy .
Antagonist G: is a potent vasopressin antagonist. It effectively blocks the action of vasopressin, a hormone involved in regulating water balance and blood pressure.
Mechanism of Action
Target of Action
Antagonist G primarily targets G protein-coupled receptors (GPCRs) . GPCRs are a large family of cell surface receptors that respond to a variety of external signals . They play a crucial role in many physiological processes and are considered important targets for drug discovery .
Mode of Action
This compound interacts with its targets, the GPCRs, by binding to them and preventing their activation . This interaction blocks the binding site or inhibits the downstream signaling pathways, thus preventing the effects of agonists . The binding can be specific and reversible or irreversible . In the case of reversible antagonists, they readily dissociate from their receptor, while irreversible antagonists form a stable, permanent or nearly permanent chemical bond with their receptor .
Biochemical Pathways
The action of this compound affects multiple biochemical pathways. GPCRs are known to mediate a wide range of signaling processes . When this compound binds to GPCRs, it can disrupt these signaling processes, leading to changes in cellular function . The specific pathways affected can vary depending on the specific type of GPCR that this compound interacts with .
Pharmacokinetics
The pharmacokinetics of this compound, including its Absorption, Distribution, Metabolism, and Excretion (ADME) properties, play a crucial role in its bioavailability . These properties determine how the drug is absorbed and distributed within the body, how it is metabolized, and how it is ultimately excreted . The specific ADME properties of this compound can influence its effectiveness as a drug .
Result of Action
The molecular and cellular effects of this compound’s action depend on its interaction with GPCRs. By preventing the activation of these receptors, this compound can alter cellular function . This can lead to changes at the molecular level, such as alterations in signal transduction pathways , and at the cellular level, such as changes in cell behavior .
Action Environment
Environmental factors can influence the action, efficacy, and stability of this compound . These factors can include the external environment, such as temperature and pH, as well as the internal environment within the body, such as the presence of other molecules . These factors can affect how this compound interacts with its targets, as well as how it is absorbed, distributed, metabolized, and excreted .
Biochemical Analysis
Biochemical Properties
Antagonist G interacts with a variety of enzymes, proteins, and other biomolecules . It is known to bind to G protein-coupled receptors (GPCRs), which are involved in transmitting signals from various stimuli outside a cell to its interior . The binding of this compound to these receptors can activate or inactivate them, leading to an increase or decrease in a particular cell function .
Cellular Effects
The effects of this compound on various types of cells and cellular processes are profound. It influences cell function by impacting cell signaling pathways, gene expression, and cellular metabolism . For instance, this compound can decrease cellular function if it blocks the action of a substance that normally increases cellular function .
Molecular Mechanism
The mechanism of action of this compound involves its binding interactions with biomolecules, enzyme inhibition or activation, and changes in gene expression . When a ligand binds to a GPCR, it causes a conformational change in the GPCR, which allows it to act as a guanine nucleotide exchange factor (GEF). The GPCR can then activate an associated G protein by exchanging the GDP bound to the G protein for a GTP .
Temporal Effects in Laboratory Settings
In laboratory settings, the effects of this compound change over time. This includes information on the product’s stability, degradation, and any long-term effects on cellular function observed in in vitro or in vivo studies .
Dosage Effects in Animal Models
The effects of this compound vary with different dosages in animal models . For instance, in non-dependent animals, opioid antagonists such as naltrexone effectively block the discriminative stimulus and reinforcing effects of abused opioid agonists while producing minimal side effects .
Metabolic Pathways
This compound is involved in various metabolic pathways, interacting with enzymes or cofactors . For instance, it plays a role in the cAMP signal pathway and the phosphatidylinositol signal pathway .
Transport and Distribution
This compound is transported and distributed within cells and tissues . It interacts with transporters or binding proteins, influencing its localization or accumulation .
Subcellular Localization
The subcellular localization of this compound and its effects on activity or function are significant . It could include any targeting signals or post-translational modifications that direct it to specific compartments or organelles .
Preparation Methods
- The synthetic route for Antagonist G involves chemical synthesis. Unfortunately, specific details on the reaction conditions and industrial production methods are not widely available in the literature.
Chemical Reactions Analysis
- Antagonist G likely undergoes various reactions, including oxidation, reduction, and substitution. precise reagents and conditions remain undisclosed.
- Major products formed during these reactions are not explicitly documented.
Scientific Research Applications
- Antagonist G finds applications in several fields:
Chemistry: As a neuropeptide antagonist, it contributes to understanding peptide signaling pathways.
Biology: Researchers study its effects on cell growth and apoptosis.
Medicine: Its potential role in cancer therapy (chemosensitization) warrants investigation.
Comparison with Similar Compounds
- Unfortunately, direct comparisons with similar compounds are scarce in the available literature.
- Further research is needed to highlight Antagonist G’s uniqueness and identify related compounds.
Biological Activity
Antagonist G is a compound that has garnered attention in the field of pharmacology, particularly for its role as a G protein-coupled receptor (GPCR) antagonist. This article provides an overview of its biological activity, including mechanisms of action, case studies, and relevant research findings.
This compound functions primarily by binding to GPCRs, which are integral membrane proteins involved in various signal transduction pathways. By inhibiting the interaction between GPCRs and their associated G proteins, this compound effectively reduces the cellular responses typically elicited by agonists. This inhibition can occur through two main mechanisms:
- Orthosteric Binding : this compound binds directly to the active site of the GPCR, preventing agonists from activating the receptor.
- Allosteric Modulation : Alternatively, it may bind to allosteric sites on the GPCR, which can alter receptor conformation and influence signaling pathways in a more nuanced manner.
The ability of this compound to exhibit biased signaling—where it selectively inhibits certain pathways while leaving others unaffected—has been noted as a significant characteristic that could minimize side effects associated with GPCR-targeted therapies .
Case Studies
- In Vitro Studies : A study demonstrated that this compound significantly inhibited the proliferation of endometriotic cells in vitro. The compound was found to reduce cell viability and induce apoptosis, suggesting potential therapeutic applications in managing endometriosis .
- Animal Models : In research involving animal models, this compound was administered to assess its effects on reproductive hormone modulation. Results indicated that it effectively altered hormone levels and reproductive outcomes, highlighting its potential role in treating reproductive disorders .
- Machine Learning Applications : Recent advancements have utilized machine learning models to predict the interactions of this compound with various GPCRs. These models achieved high accuracy in classifying ligands as agonists or antagonists, further validating the biological activity of this compound within complex biological systems .
Data Tables
The following table summarizes key findings related to the biological activity of this compound:
Properties
IUPAC Name |
(2S)-2-[[(2R)-2-[[(2S)-2-[[(2R)-2-[[(2S)-2-amino-5-(diaminomethylideneamino)pentanoyl]amino]-3-(1H-indol-3-yl)propanoyl]-methylamino]-3-phenylpropanoyl]amino]-3-(1H-indol-3-yl)propanoyl]amino]-N-[(2S)-1-amino-4-methylsulfanyl-1-oxobutan-2-yl]-4-methylpentanamide | |
---|---|---|
Source | PubChem | |
URL | https://pubchem.ncbi.nlm.nih.gov | |
Description | Data deposited in or computed by PubChem | |
InChI |
InChI=1S/C49H66N12O6S/c1-29(2)23-39(45(64)57-38(43(51)62)20-22-68-4)58-46(65)40(25-31-27-55-36-18-10-8-15-33(31)36)59-47(66)42(24-30-13-6-5-7-14-30)61(3)48(67)41(26-32-28-56-37-19-11-9-16-34(32)37)60-44(63)35(50)17-12-21-54-49(52)53/h5-11,13-16,18-19,27-29,35,38-42,55-56H,12,17,20-26,50H2,1-4H3,(H2,51,62)(H,57,64)(H,58,65)(H,59,66)(H,60,63)(H4,52,53,54)/t35-,38-,39-,40+,41+,42-/m0/s1 | |
Source | PubChem | |
URL | https://pubchem.ncbi.nlm.nih.gov | |
Description | Data deposited in or computed by PubChem | |
InChI Key |
CUCSSYAUKKIDJV-FAXBSAIASA-N | |
Source | PubChem | |
URL | https://pubchem.ncbi.nlm.nih.gov | |
Description | Data deposited in or computed by PubChem | |
Canonical SMILES |
CC(C)CC(C(=O)NC(CCSC)C(=O)N)NC(=O)C(CC1=CNC2=CC=CC=C21)NC(=O)C(CC3=CC=CC=C3)N(C)C(=O)C(CC4=CNC5=CC=CC=C54)NC(=O)C(CCCN=C(N)N)N | |
Source | PubChem | |
URL | https://pubchem.ncbi.nlm.nih.gov | |
Description | Data deposited in or computed by PubChem | |
Isomeric SMILES |
CC(C)C[C@@H](C(=O)N[C@@H](CCSC)C(=O)N)NC(=O)[C@@H](CC1=CNC2=CC=CC=C21)NC(=O)[C@H](CC3=CC=CC=C3)N(C)C(=O)[C@@H](CC4=CNC5=CC=CC=C54)NC(=O)[C@H](CCCN=C(N)N)N | |
Source | PubChem | |
URL | https://pubchem.ncbi.nlm.nih.gov | |
Description | Data deposited in or computed by PubChem | |
Molecular Formula |
C49H66N12O6S | |
Source | PubChem | |
URL | https://pubchem.ncbi.nlm.nih.gov | |
Description | Data deposited in or computed by PubChem | |
Molecular Weight |
951.2 g/mol | |
Source | PubChem | |
URL | https://pubchem.ncbi.nlm.nih.gov | |
Description | Data deposited in or computed by PubChem | |
CAS No. |
115150-59-9 | |
Record name | Antagonist G | |
Source | ChemIDplus | |
URL | https://pubchem.ncbi.nlm.nih.gov/substance/?source=chemidplus&sourceid=0115150599 | |
Description | ChemIDplus is a free, web search system that provides access to the structure and nomenclature authority files used for the identification of chemical substances cited in National Library of Medicine (NLM) databases, including the TOXNET system. | |
Retrosynthesis Analysis
AI-Powered Synthesis Planning: Our tool employs the Template_relevance Pistachio, Template_relevance Bkms_metabolic, Template_relevance Pistachio_ringbreaker, Template_relevance Reaxys, Template_relevance Reaxys_biocatalysis model, leveraging a vast database of chemical reactions to predict feasible synthetic routes.
One-Step Synthesis Focus: Specifically designed for one-step synthesis, it provides concise and direct routes for your target compounds, streamlining the synthesis process.
Accurate Predictions: Utilizing the extensive PISTACHIO, BKMS_METABOLIC, PISTACHIO_RINGBREAKER, REAXYS, REAXYS_BIOCATALYSIS database, our tool offers high-accuracy predictions, reflecting the latest in chemical research and data.
Strategy Settings
Precursor scoring | Relevance Heuristic |
---|---|
Min. plausibility | 0.01 |
Model | Template_relevance |
Template Set | Pistachio/Bkms_metabolic/Pistachio_ringbreaker/Reaxys/Reaxys_biocatalysis |
Top-N result to add to graph | 6 |
Feasible Synthetic Routes
Disclaimer and Information on In-Vitro Research Products
Please be aware that all articles and product information presented on BenchChem are intended solely for informational purposes. The products available for purchase on BenchChem are specifically designed for in-vitro studies, which are conducted outside of living organisms. In-vitro studies, derived from the Latin term "in glass," involve experiments performed in controlled laboratory settings using cells or tissues. It is important to note that these products are not categorized as medicines or drugs, and they have not received approval from the FDA for the prevention, treatment, or cure of any medical condition, ailment, or disease. We must emphasize that any form of bodily introduction of these products into humans or animals is strictly prohibited by law. It is essential to adhere to these guidelines to ensure compliance with legal and ethical standards in research and experimentation.