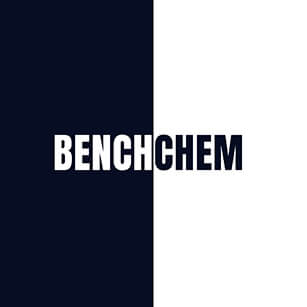
ML 233
- Click on QUICK INQUIRY to receive a quote from our team of experts.
- With the quality product at a COMPETITIVE price, you can focus more on your research.
Description
Non-peptide apelin receptor agonist (EC50 = 3.7 μM); exhibits >21-fold selectivity over the closely related angiotensin 1 receptor (EC50 = >79 μM).
Scientific Research Applications
Machine Learning in Physical Sciences
Machine learning (ML) has significantly permeated various scientific disciplines, including the physical sciences. Its applications span a multitude of domains such as particle physics, cosmology, quantum many-body physics, quantum computing, and chemical and material physics. The collaboration between ML and physical sciences has led to conceptual advancements in ML, inspired by physical insights, and vice versa. This synergy has not only facilitated a deeper understanding of physical phenomena but also accelerated advancements in computational techniques, showcasing the multifaceted benefits of ML in scientific exploration (Carleo et al., 2019).
Machine Learning in Nuclear Data Evaluation
Machine learning has also proven to be instrumental in the field of nuclear data evaluation. The predictive capabilities of ML models have been successfully employed to infer neutron-induced reaction cross-sections, demonstrating superior accuracy compared to traditional physics-guided models. This approach mitigates human bias, reduces uncertainties, and enables faster, more reliable decision-making in nuclear data evaluation. The integration of ML in this domain exemplifies its potential to refine and expedite complex evaluative tasks (Vicente-Valdez et al., 2021).
Machine Learning in Seismology
In seismology, ML has emerged as a transformative force, enabling the identification of unseen signals and patterns, and enhancing the understanding of seismic phenomena. Applications range from earthquake detection and early warning systems to ground-motion prediction and seismic tomography. The adoption of ML in seismology underscores its capability to amalgamate data-driven insights with traditional physical modeling, paving the way for comprehensive seismic analysis and prediction (Kong et al., 2018).
Machine Learning in Cardiac Electrophysiology and Arrhythmias
ML's application extends to the field of cardiac electrophysiology and arrhythmias, where it offers novel pathways for understanding arrhythmia mechanisms and improving the techniques for mapping cardiac electrical function. The versatility of ML in analyzing large datasets and identifying key features is particularly beneficial in this domain, facilitating the development of predictive models and aiding in decision-making for arrhythmia management (Trayanova et al., 2021).
Properties
Molecular Formula |
C19H21NO4S |
---|---|
Molecular Weight |
359.44 |
Synonyms |
(E)-4-Methyl-5-(((phenylsulfonyl)oxy)imino)-[1,1/'-bi(cyclohexane)]-3,6-dien-2-one |
Origin of Product |
United States |
Disclaimer and Information on In-Vitro Research Products
Please be aware that all articles and product information presented on BenchChem are intended solely for informational purposes. The products available for purchase on BenchChem are specifically designed for in-vitro studies, which are conducted outside of living organisms. In-vitro studies, derived from the Latin term "in glass," involve experiments performed in controlled laboratory settings using cells or tissues. It is important to note that these products are not categorized as medicines or drugs, and they have not received approval from the FDA for the prevention, treatment, or cure of any medical condition, ailment, or disease. We must emphasize that any form of bodily introduction of these products into humans or animals is strictly prohibited by law. It is essential to adhere to these guidelines to ensure compliance with legal and ethical standards in research and experimentation.