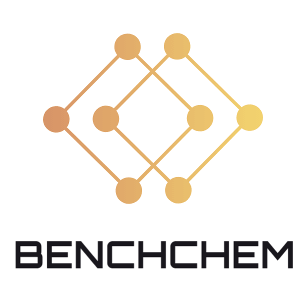
ML400
- 専門家チームからの見積もりを受け取るには、QUICK INQUIRYをクリックしてください。
- 品質商品を競争力のある価格で提供し、研究に集中できます。
説明
ML400 is an allosteric inhibitor of LMPTP. This compound. This compound is potent (EC50∼1μM), selective against other phosphatases, and displays good cell-based activity as well as rodent pharmacokinetics. As such it should be a valuable tool to explore the effects of selective LMPTP inhibition in vitro and in vivo.
科学的研究の応用
Neurosurgical Care
Machine learning (ML) has shown significant potential in improving neurosurgical care by enhancing the efficiency and precision of perioperative decision-making. ML models in neurosurgery assist in surgical treatment planning, intraoperative guidance, neurophysiological monitoring, and outcome prediction for various conditions like epilepsy, brain tumors, spinal lesions, neurovascular pathology, Parkinson’s disease, traumatic brain injury, and hydrocephalus. However, it's crucial to thoroughly validate specific ML models before their implementation in clinical neurosurgical care, considering practical and ethical issues parallel to the development of these techniques (Senders et al., 2017).
Science Assessment
ML is revolutionizing science assessment by enabling complex assessments to be used by teachers without the burden of human scoring, thereby saving time and cost. Most studies in this domain involve text recognition, classification, and scoring with a focus on constructing scientific explanations. Supervised ML, relying on attribute extraction from student work coded by humans, is the most common, emphasizing the construction of scientific explanations (Zhai et al., 2020).
Energy Economics and Finance
ML is creating new opportunities for innovative research in energy economics and finance, with applications in predicting energy prices, demand forecasting, risk management, trading strategies, data processing, and analyzing macro/energy trends. Support Vector Machine (SVM), Artificial Neural Network (ANN), and Genetic Algorithms (GAs) are among the most popular techniques used in this field, but the literature also points out certain limitations and gaps that future research needs to address (Ghoddusi et al., 2019).
Cardiovascular Imaging
ML is increasingly used in cardiology, particularly in cardiovascular imaging. It aids investigators, data scientists, authors, editors, and reviewers involved in ML research, ensuring uniform reporting of ML investigations. However, due to the complexity and flexibility of ML algorithms, inconsistencies in model performance and interpretation may occur. A comprehensive list of crucial responsibilities needs to be completed when developing ML models to reduce algorithmic errors and biases (Sengupta et al., 2020).
Food Safety
ML has proven to be useful for monitoring and predicting food safety, with applications in Bayesian networks, Neural networks, and Support vector machines. The studies in this domain have shown high prediction accuracy by the validation process, identifying avenues for future studies applying ML models for monitoring and prediction of food safety (Wang et al., 2021).
Animal Behaviour Studies
ML methodologies are ideally suited for analyzing complex behavioral data in animal behavior research, addressing the challenge of analyzing large, detailed datasets exhibiting nonlinear dependencies and unknown interactions across multiple variables. ML techniques allow for the extraction of knowledge from these data, playing a central role in translating data into scientific knowledge (Valletta et al., 2017).
特性
CAS番号 |
1908414-42-5 |
---|---|
分子式 |
C24H29N3O |
分子量 |
375.516 |
IUPAC名 |
2-(4-Methoxyphenyl)-N-(3-piperidin-1-ylpropyl)quinolin-4-amine |
InChI |
InChI=1S/C24H29N3O/c1-28-20-12-10-19(11-13-20)23-18-24(21-8-3-4-9-22(21)26-23)25-14-7-17-27-15-5-2-6-16-27/h3-4,8-13,18H,2,5-7,14-17H2,1H3,(H,25,26) |
InChIキー |
CCEQOVGPLKQKET-UHFFFAOYSA-N |
SMILES |
COC1=CC=C(C2=NC3=CC=CC=C3C(NCCCN4CCCCC4)=C2)C=C1 |
外観 |
Solid powder |
純度 |
>98% (or refer to the Certificate of Analysis) |
賞味期限 |
>3 years if stored properly |
溶解性 |
Soluble in DMSO |
保存方法 |
Dry, dark and at 0 - 4 C for short term (days to weeks) or -20 C for long term (months to years). |
同義語 |
ML400; ML-400; ML 400; CID 73050863; CID-73050863; CID73050863; SID 173019983; SID-173019983; SID173019983; |
製品の起源 |
United States |
Retrosynthesis Analysis
AI-Powered Synthesis Planning: Our tool employs the Template_relevance Pistachio, Template_relevance Bkms_metabolic, Template_relevance Pistachio_ringbreaker, Template_relevance Reaxys, Template_relevance Reaxys_biocatalysis model, leveraging a vast database of chemical reactions to predict feasible synthetic routes.
One-Step Synthesis Focus: Specifically designed for one-step synthesis, it provides concise and direct routes for your target compounds, streamlining the synthesis process.
Accurate Predictions: Utilizing the extensive PISTACHIO, BKMS_METABOLIC, PISTACHIO_RINGBREAKER, REAXYS, REAXYS_BIOCATALYSIS database, our tool offers high-accuracy predictions, reflecting the latest in chemical research and data.
Strategy Settings
Precursor scoring | Relevance Heuristic |
---|---|
Min. plausibility | 0.01 |
Model | Template_relevance |
Template Set | Pistachio/Bkms_metabolic/Pistachio_ringbreaker/Reaxys/Reaxys_biocatalysis |
Top-N result to add to graph | 6 |
Feasible Synthetic Routes
試験管内研究製品の免責事項と情報
BenchChemで提示されるすべての記事および製品情報は、情報提供を目的としています。BenchChemで購入可能な製品は、生体外研究のために特別に設計されています。生体外研究は、ラテン語の "in glass" に由来し、生物体の外で行われる実験を指します。これらの製品は医薬品または薬として分類されておらず、FDAから任何の医療状態、病気、または疾患の予防、治療、または治癒のために承認されていません。これらの製品を人間または動物に体内に導入する形態は、法律により厳格に禁止されています。これらのガイドラインに従うことは、研究と実験において法的および倫理的な基準の遵守を確実にするために重要です。