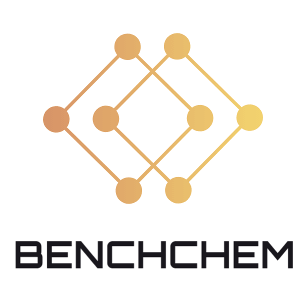
CO1686-DA
- Click on QUICK INQUIRY to receive a quote from our team of experts.
- With the quality product at a COMPETITIVE price, you can focus more on your research.
Overview
Description
CO1686-DA, is a CO1686 precursor, also a des-acryl analogue of CO1686. CO1686 is an orally available small molecule, irreversible inhibitor of epidermal growth factor receptor (EGFR) with potential antineoplastic activity. EGFR inhibitor CO1686 binds to and inhibits mutant forms of EGFR, including T790M, thereby leading to cell death of resistant tumor cells. Compared to other EGFR inhibitors, CO1686 inhibits T790M, a secondary acquired resistance mutation, as well as other mutant EGFRs and may have therapeutic benefits in tumors with T790M-mediated resistance to other EGFR tyrosine kinase inhibitors. This agent shows minimal activity against wild-type EGFR, hence does not cause certain dose-limiting toxicities.
Scientific Research Applications
Enhancing Programming Productivity in Scientific Research
Appelbe, Moresi, Quenette, and Simter (2007) discussed the development and use of scientific software frameworks, which are crucial in improving programming productivity in scientific research. These frameworks are essential for grid-enabling existing applications and developing new applications, particularly in traditional programming languages such as C and Fortran, which are commonly used in scientific research (Appelbe, Moresi, Quenette, & Simter, 2007).
Collaborative Online Visualization and Steering in Computational Grids
Riedel et al. (2007) evaluated the Collaborative Online Visualization and Steering (COVS) Framework in Grids. This framework is significant in scientific research for performing simulations and visualizations simultaneously, thus increasing the efficiency of e-Scientists in large-scale scientific research projects (Riedel et al., 2007).
Assessing Scientific Reasoning
Zhou et al. (2016) focused on assessing student reasoning in control of variables, a core sub-skill of scientific reasoning. Their study provides insights into the impact of experimental data on student performance and reasoning in scientific contexts, contributing to the development of effective scientific research methodologies (Zhou et al., 2016).
Human Computing Integration in Scientific Research
Clark, Greenberg, and Ouellette (2018) proposed a model for integrating Human Computing into commercial video games, highlighting the potential for this approach in accelerating scientific research, particularly in the field of chemotherapeutic co-medication for cancer research (Clark, Greenberg, & Ouellette, 2018).
Designing and Evaluating Scientific Workflows for Big Data
Etemadpour et al. (2015) explored the design and evaluation of scientific workflows in research-oriented web applications, focusing on the analysis of large, heterogeneous scientific datasets. This research contributes to improving user experience and efficiency in scientific data analysis (Etemadpour et al., 2015).
Co-Evolution of Scientific and Technological Networks in Biomedicine
Murray (2002) examined the co-evolution of scientific and technological networks in biomedicine, specifically in the context of tissue engineering. This study highlights the dynamics of knowledge transfer and commercialization in scientific research (Murray, 2002).
Blockchain-Based Scientific Publishing
Mackey et al. (2019) proposed a governance framework for scientific publishing based on a consortium blockchain model. This framework aims to increase transparency and efficiency in the scientific publishing process, leveraging blockchain technology to facilitate research data provenance and optimize peer-review processes (Mackey et al., 2019).
Accelerating Scientific Discoveries through Hackathons
Ghouila et al. (2018) demonstrated how hackathons can accelerate scientific discoveries and knowledge transfer. They emphasized the role of hackathons in enhancing collaborative science and driving reproducibility of scientific analyses (Ghouila et al., 2018).
Data-Driven Manipulation of 3-D Heterogeneous Compliant Objects
Alambeigi et al. (2018) presented a data-driven method for manipulating 3-D compliant objects with heterogeneous physical properties, relevant for various medical and industrial applications in scientific research (Alambeigi, Wang, Hegeman, Liu, & Armand, 2018).
Child-Led Enquiry in Primary Science
Dunlop et al. (2013) explored the application of a child-led approach to scientific enquiry, demonstrating its effectiveness in engaging children with science learning and developing confidence and oracy skills, contributing to the pedagogical strategies in scientific education (Dunlop, Compton, Clarke, & McKelvey-Martin, 2013).
properties
Molecular Formula |
C24H26F3N7O2 |
---|---|
Molecular Weight |
501.5 |
IUPAC Name |
1-(4-(4-((4-((3-aminophenyl)amino)-5-(trifluoromethyl)pyrimidin-2-yl)amino)-3-methoxyphenyl)piperazin-1-yl)ethanone |
InChI |
InChI=1S/C24H26F3N7O2/c1-15(35)33-8-10-34(11-9-33)18-6-7-20(21(13-18)36-2)31-23-29-14-19(24(25,26)27)22(32-23)30-17-5-3-4-16(28)12-17/h3-7,12-14H,8-11,28H2,1-2H3,(H2,29,30,31,32) |
InChI Key |
PXQZEXVGMRJEQE-UHFFFAOYSA-N |
SMILES |
NC1=CC=CC(NC2=NC(NC3=CC=C(N4CCN(C(C)=O)CC4)C=C3OC)=NC=C2C(F)(F)F)=C1 |
Appearance |
white solid powder |
Purity |
>98% (or refer to the Certificate of Analysis) |
shelf_life |
>2 years if stored properly |
solubility |
Soluble in DMSO, not in water |
storage |
Dry, dark and at 0 - 4 C for short term (days to weeks) or -20 C for long term (months to years). |
synonyms |
CO1686 DA; CO1686DA; desacryl analogue of CO1686; CO1686 precursor. |
Origin of Product |
United States |
Retrosynthesis Analysis
AI-Powered Synthesis Planning: Our tool employs the Template_relevance Pistachio, Template_relevance Bkms_metabolic, Template_relevance Pistachio_ringbreaker, Template_relevance Reaxys, Template_relevance Reaxys_biocatalysis model, leveraging a vast database of chemical reactions to predict feasible synthetic routes.
One-Step Synthesis Focus: Specifically designed for one-step synthesis, it provides concise and direct routes for your target compounds, streamlining the synthesis process.
Accurate Predictions: Utilizing the extensive PISTACHIO, BKMS_METABOLIC, PISTACHIO_RINGBREAKER, REAXYS, REAXYS_BIOCATALYSIS database, our tool offers high-accuracy predictions, reflecting the latest in chemical research and data.
Strategy Settings
Precursor scoring | Relevance Heuristic |
---|---|
Min. plausibility | 0.01 |
Model | Template_relevance |
Template Set | Pistachio/Bkms_metabolic/Pistachio_ringbreaker/Reaxys/Reaxys_biocatalysis |
Top-N result to add to graph | 6 |
Feasible Synthetic Routes
Disclaimer and Information on In-Vitro Research Products
Please be aware that all articles and product information presented on BenchChem are intended solely for informational purposes. The products available for purchase on BenchChem are specifically designed for in-vitro studies, which are conducted outside of living organisms. In-vitro studies, derived from the Latin term "in glass," involve experiments performed in controlled laboratory settings using cells or tissues. It is important to note that these products are not categorized as medicines or drugs, and they have not received approval from the FDA for the prevention, treatment, or cure of any medical condition, ailment, or disease. We must emphasize that any form of bodily introduction of these products into humans or animals is strictly prohibited by law. It is essential to adhere to these guidelines to ensure compliance with legal and ethical standards in research and experimentation.