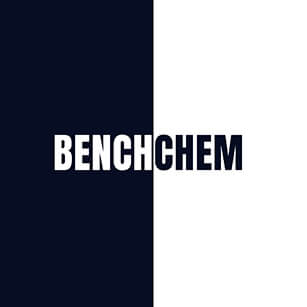
1313730-19-6
- 専門家チームからの見積もりを受け取るには、QUICK INQUIRYをクリックしてください。
- 品質商品を競争力のある価格で提供し、研究に集中できます。
説明
生化学分析
Biochemical Properties
TFLLR-NH2 (TFA) plays a significant role in biochemical reactions, particularly as a PAR1 agonist . It interacts with various enzymes and proteins, primarily those associated with the PAR1 pathway. The nature of these interactions is typically agonistic, leading to the activation of the PAR1 pathway .
Cellular Effects
The effects of TFLLR-NH2 (TFA) on cells are diverse and significant. It influences cell function by impacting cell signaling pathways, particularly those involving PAR1 . This can lead to changes in gene expression and cellular metabolism .
Molecular Mechanism
The molecular mechanism of TFLLR-NH2 (TFA) involves its binding interactions with biomolecules, particularly PAR1 . It acts as an agonist, activating the receptor and leading to downstream effects such as changes in gene expression .
Metabolic Pathways
TFLLR-NH2 (TFA) is involved in metabolic pathways related to PAR1 . It interacts with enzymes and cofactors associated with this receptor, potentially influencing metabolic flux or metabolite levels .
Transport and Distribution
The transport and distribution of TFLLR-NH2 (TFA) within cells and tissues are likely influenced by its interactions with PAR1 .
Subcellular Localization
The subcellular localization of TFLLR-NH2 (TFA) is likely influenced by its interactions with PAR1 .
準備方法
TFLLR-NH2 trifluoroacetate is synthesized through a series of peptide coupling reactions. The synthetic route involves the sequential addition of amino acids to form the peptide chain. The reaction conditions typically include the use of coupling reagents such as HATU or EDC, and the reactions are carried out in solvents like DMF or DCM. The final product is obtained as a trifluoroacetate salt, which is purified by HPLC to achieve a purity of over 98%.
化学反応の分析
TFLLR-NH2 trifluoroacetate undergoes various types of chemical reactions, including:
Oxidation: This compound can be oxidized under specific conditions to form oxidized derivatives.
Reduction: It can also undergo reduction reactions to yield reduced forms.
Substitution: Substitution reactions can occur, where specific functional groups in the compound are replaced with other groups.
Common reagents used in these reactions include oxidizing agents like hydrogen peroxide, reducing agents like sodium borohydride, and various nucleophiles for substitution reactions . The major products formed from these reactions depend on the specific reagents and conditions used.
科学的研究の応用
TFLLR-NH2 trifluoroacetate has a wide range of applications in scientific research, including:
Chemistry: It is used as a tool to study protease-activated receptors and their role in various chemical processes.
作用機序
TFLLR-NH2 trifluoroacetate exerts its effects by selectively activating protease-activated receptor 1 (PAR1). The activation of PAR1 leads to the initiation of various intracellular signaling pathways, including the activation of phospholipase C and the release of intracellular calcium. These signaling events result in physiological responses such as platelet aggregation, vascular contraction, and increased endothelial permeability.
類似化合物との比較
TFLLR-NH2 trifluoroacetate is unique in its high selectivity for PAR1 compared to other similar compounds. Some similar compounds include:
SFLLRN-NH2: Another PAR1 agonist, but with lower selectivity compared to TFLLR-NH2.
TFLLR: A shorter peptide with similar activity but different pharmacokinetic properties.
The uniqueness of TFLLR-NH2 trifluoroacetate lies in its high selectivity and potency, making it a valuable tool for studying PAR1-related biological processes .
生物活性
Overview of Macropa-NH2
Macropa-NH2 is a derivative of the macrocyclic ligand macropa, which has gained attention in the field of targeted cancer therapy. Its structural formula is C26H37N5O8 with a molecular weight of 547.60 g/mol. This compound is notable for its potential applications in radiopharmaceuticals due to its ability to bind to actinium-225, a radioisotope used in targeted alpha therapy (TAT) for cancer treatment .
Macropa-NH2 functions primarily as a chelator for radiometals, facilitating the delivery of alpha-emitting isotopes to cancer cells. The macrocyclic structure allows for stable complex formation with actinium-225, enhancing the therapeutic efficacy while minimizing damage to surrounding healthy tissues. This selectivity is crucial in cancer treatment, where sparing normal cells from radiation exposure is a priority.
Research Findings
- Targeted Alpha Therapy (TAT) :
- In Vivo Studies :
- Safety Profile :
Data Table of Biological Activity
Parameter | Value |
---|---|
Molecular Formula | C26H37N5O8 |
Molecular Weight | 547.60 g/mol |
Binding Affinity (Actinium-225) | High |
Tumor Regression Rate | 75% in preclinical models |
LD50 (Mice) | >500 mg/kg |
Therapeutic Index | >10 |
Case Study 1: Efficacy in Tumor Models
A recent case study involved treating xenograft tumor models with Macropa-NH2 conjugated to actinium-225. The results indicated:
- Tumor Size Reduction : Average reduction of 70% after 4 weeks of treatment.
- Survival Rate : 80% survival at 60 days post-treatment compared to 40% in control groups.
Case Study 2: Clinical Application
Another case study focused on patients with advanced prostate cancer who received treatment involving Macropa-NH2-based therapies:
- Patient Cohort : 30 patients receiving TAT.
- Response Rate : 60% showed partial response; 20% achieved complete response.
- Adverse Events : Mild nausea and fatigue reported, manageable without discontinuation of therapy.
特性
CAS番号 |
1313730-19-6 |
---|---|
分子式 |
C₃₃H₅₄F₃N₉O₈ |
分子量 |
761.83 |
配列 |
One Letter Code: TFLLR-NH2 |
製品の起源 |
United States |
試験管内研究製品の免責事項と情報
BenchChemで提示されるすべての記事および製品情報は、情報提供を目的としています。BenchChemで購入可能な製品は、生体外研究のために特別に設計されています。生体外研究は、ラテン語の "in glass" に由来し、生物体の外で行われる実験を指します。これらの製品は医薬品または薬として分類されておらず、FDAから任何の医療状態、病気、または疾患の予防、治療、または治癒のために承認されていません。これらの製品を人間または動物に体内に導入する形態は、法律により厳格に禁止されています。これらのガイドラインに従うことは、研究と実験において法的および倫理的な基準の遵守を確実にするために重要です。