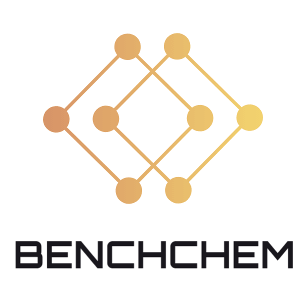
EHT-6706
- 専門家チームからの見積もりを受け取るには、QUICK INQUIRYをクリックしてください。
- 品質商品を競争力のある価格で提供し、研究に集中できます。
説明
EHT-6706 is a novel microtubule-disrupting agent that targets the colchicine-binding site to inhibit tubulin polymerization. At low nM concentrations, EHT 6706 exhibits highly potent antiproliferative activity on more than 60 human tumor cell lines, even those described as being drug resistant. EHT 6706 also shows strong efficacy as a vascular-disrupting agent, since it prevents endothelial cell tube formation and disrupts pre-established vessels, changes the permeability of endothelial cell monolayers and inhibits endothelial cell migration. Genome-wide transcriptomic analysis of EHT 6706 effects on human endothelial cells shows that the antiangiogenic activity elicits gene deregulations of antiangiogenic pathways. These findings indicate that EHT 6706 is a promising tubulin-binding compound with potentially broad clinical antitumor efficacy.
科学的研究の応用
Enhancing Reproducibility of Biomedical Research
Reproducibility in Biomedical Research Using EHRs The reproducibility of biomedical research findings is crucial for validation and evaluation by the broader community. Research using electronic health records (EHRs) often faces reproducibility challenges due to inadequate sharing of data preprocessing, cleaning, phenotyping, and analysis details. With increasing complexity and volume of EHR data sources, ensuring reproducibility and transparency in scientific findings derived from EHR data is paramount. Adopting scientific software engineering principles, source code revision control systems, and standardizing common data management and analytical approaches can significantly enhance the reproducibility of research using EHRs (Denaxas et al., 2017).
Machine Learning in Diabetes Research
Machine Learning and Data Mining in Diabetes Research The explosion of data in biotechnology and health sciences, including genetic and EHR data, necessitates the application of machine learning and data mining methods in biosciences. Specifically, in diabetes research, machine learning and data mining have been employed for prediction and diagnosis, understanding diabetic complications, exploring genetic backgrounds, and optimizing health care and management. Support vector machines (SVM) are highlighted as a particularly successful algorithm in this domain (Kavakiotis et al., 2017).
Population Health Research with EHRs
Utilizing EHRs for Population Health Research EHRs, beyond their clinical utility, offer a rich source for epidemiologic investigations and population health research. The wide range of research applications benefiting from the large sample sizes and generalizable patient populations provided by EHRs include reevaluation of prior findings, exploration of various diseases, environmental and social epidemiology, and evaluation of natural experiments. Despite the inherent advantages, there are challenges like data reliability and population retention compared to primary data collection methods. However, the lower cost and shorter time required for EHR-based studies make them an attractive option for population health research (Casey et al., 2016).
Clinical Information Extraction from EHRs
Information Extraction from EHRs The rapid adoption of EHRs presents an opportunity to extract and encode clinical information to support automated systems and enable secondary use of EHR data for clinical and translational research. The information extraction task is critical for facilitating the secondary use of EHR data, and a variety of clinical information extraction applications have been employed in disease- and drug-related studies and clinical workflow optimizations. However, there's a noticeable gap between clinical studies using EHR data and those utilizing clinical information extraction, underscoring the potential to bridge this gap for enhanced research outcomes (Wang et al., 2018).
特性
CAS番号 |
1351592-10-3 |
---|---|
分子式 |
C21H26Cl2N2O4 |
分子量 |
441.34814 |
IUPAC名 |
7-ethoxy-4-(3,4,5-trimethoxybenzyl)isoquinolin-8-amine dihydrochloride |
InChI |
InChI=1S/C21H24N2O4.2ClH/c1-5-27-17-7-6-15-14(11-23-12-16(15)20(17)22)8-13-9-18(24-2)21(26-4)19(10-13)25-3;;/h6-7,9-12H,5,8,22H2,1-4H3;2*1H |
InChIキー |
VGHUBZMBLUZIJE-UHFFFAOYSA-N |
SMILES |
NC1=C(OCC)C=CC2=C1C=NC=C2CC3=CC(OC)=C(OC)C(OC)=C3.[H]Cl.[H]Cl |
外観 |
Solid powder |
純度 |
>98% (or refer to the Certificate of Analysis) |
賞味期限 |
>5 years if stored properly |
溶解性 |
Soluble in DMSO, not in water |
保存方法 |
Dry, dark and at 0 - 4 C for short term (days to weeks) or -20 C for long term (months to years). |
同義語 |
EHT6706; EHT 6706; EHT-6706. |
製品の起源 |
United States |
Retrosynthesis Analysis
AI-Powered Synthesis Planning: Our tool employs the Template_relevance Pistachio, Template_relevance Bkms_metabolic, Template_relevance Pistachio_ringbreaker, Template_relevance Reaxys, Template_relevance Reaxys_biocatalysis model, leveraging a vast database of chemical reactions to predict feasible synthetic routes.
One-Step Synthesis Focus: Specifically designed for one-step synthesis, it provides concise and direct routes for your target compounds, streamlining the synthesis process.
Accurate Predictions: Utilizing the extensive PISTACHIO, BKMS_METABOLIC, PISTACHIO_RINGBREAKER, REAXYS, REAXYS_BIOCATALYSIS database, our tool offers high-accuracy predictions, reflecting the latest in chemical research and data.
Strategy Settings
Precursor scoring | Relevance Heuristic |
---|---|
Min. plausibility | 0.01 |
Model | Template_relevance |
Template Set | Pistachio/Bkms_metabolic/Pistachio_ringbreaker/Reaxys/Reaxys_biocatalysis |
Top-N result to add to graph | 6 |
Feasible Synthetic Routes
試験管内研究製品の免責事項と情報
BenchChemで提示されるすべての記事および製品情報は、情報提供を目的としています。BenchChemで購入可能な製品は、生体外研究のために特別に設計されています。生体外研究は、ラテン語の "in glass" に由来し、生物体の外で行われる実験を指します。これらの製品は医薬品または薬として分類されておらず、FDAから任何の医療状態、病気、または疾患の予防、治療、または治癒のために承認されていません。これらの製品を人間または動物に体内に導入する形態は、法律により厳格に禁止されています。これらのガイドラインに従うことは、研究と実験において法的および倫理的な基準の遵守を確実にするために重要です。