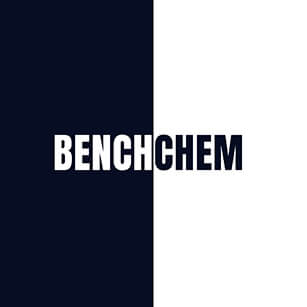
ML372
- 専門家チームからの見積もりを受け取るには、QUICK INQUIRYをクリックしてください。
- 品質商品を競争力のある価格で提供し、研究に集中できます。
説明
ML372 is a potent and selective SMN Modulator (EC50 = 12 nM, 325% increase inSMN2). This compound Increases SMN Protein Abundance, Body Weight, Lifespan, and Rescues Motor Function in SMNΔ7 SMA Mice. possessed a more stable half-life in mouse liver microsomes (T1/2 > 60 min) and high permeability in Caco-2 cells (Papp A to B = 33.8 × 10−6 cms−1 and Papp B to A = 44.1 × 10−6 cms−1) with an efflux ratio close to unity (i.e., 1.3). This compound shows good potency, pharmacokinetics, tolerance, and CNS penetration that are able to increase levels of SMN protein in several model cell lines. Spinal muscular atrophy (SMA) is an autosomal recessive neurodegenerative disease and the most common inherited cause of infant mortality. SMA, with a carrier frequency of ∼1:40, affects ∼ 1:8,000 births.
特性
CAS番号 |
1331745-61-9 |
---|---|
分子式 |
C18H20N2O4S |
分子量 |
360.428 |
IUPAC名 |
1-(5-(3,4-dihydro-2H-benzo[b][1,4]dioxepin-7-yl)thiazol-2-yl)piperidine-4-carboxylic acid |
InChI |
InChI=1S/C18H20N2O4S/c21-17(22)12-4-6-20(7-5-12)18-19-11-16(25-18)13-2-3-14-15(10-13)24-9-1-8-23-14/h2-3,10-12H,1,4-9H2,(H,21,22) |
InChIキー |
HAVNRFQWAXTDTI-UHFFFAOYSA-N |
SMILES |
O=C(O)C(CC1)CCN1C2=NC=C(S2)C3=CC(OCCCO4)=C4C=C3 |
外観 |
Solid powder |
純度 |
>98% (or refer to the Certificate of Analysis) |
賞味期限 |
>2 years if stored properly |
溶解性 |
Soluble in DMSO |
保存方法 |
Dry, dark and at 0 - 4 C for short term (days to weeks) or -20 C for long term (months to years). |
同義語 |
ML372; ML-372; ML 372. |
製品の起源 |
United States |
Retrosynthesis Analysis
AI-Powered Synthesis Planning: Our tool employs the Template_relevance Pistachio, Template_relevance Bkms_metabolic, Template_relevance Pistachio_ringbreaker, Template_relevance Reaxys, Template_relevance Reaxys_biocatalysis model, leveraging a vast database of chemical reactions to predict feasible synthetic routes.
One-Step Synthesis Focus: Specifically designed for one-step synthesis, it provides concise and direct routes for your target compounds, streamlining the synthesis process.
Accurate Predictions: Utilizing the extensive PISTACHIO, BKMS_METABOLIC, PISTACHIO_RINGBREAKER, REAXYS, REAXYS_BIOCATALYSIS database, our tool offers high-accuracy predictions, reflecting the latest in chemical research and data.
Strategy Settings
Precursor scoring | Relevance Heuristic |
---|---|
Min. plausibility | 0.01 |
Model | Template_relevance |
Template Set | Pistachio/Bkms_metabolic/Pistachio_ringbreaker/Reaxys/Reaxys_biocatalysis |
Top-N result to add to graph | 6 |
Feasible Synthetic Routes
試験管内研究製品の免責事項と情報
BenchChemで提示されるすべての記事および製品情報は、情報提供を目的としています。BenchChemで購入可能な製品は、生体外研究のために特別に設計されています。生体外研究は、ラテン語の "in glass" に由来し、生物体の外で行われる実験を指します。これらの製品は医薬品または薬として分類されておらず、FDAから任何の医療状態、病気、または疾患の予防、治療、または治癒のために承認されていません。これらの製品を人間または動物に体内に導入する形態は、法律により厳格に禁止されています。これらのガイドラインに従うことは、研究と実験において法的および倫理的な基準の遵守を確実にするために重要です。