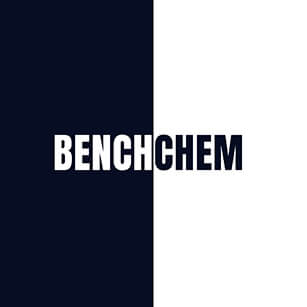
ML 233
- 専門家チームからの見積もりを受け取るには、QUICK INQUIRYをクリックしてください。
- 品質商品を競争力のある価格で提供し、研究に集中できます。
説明
Non-peptide apelin receptor agonist (EC50 = 3.7 μM); exhibits >21-fold selectivity over the closely related angiotensin 1 receptor (EC50 = >79 μM).
科学的研究の応用
Machine Learning in Physical Sciences
Machine learning (ML) has significantly permeated various scientific disciplines, including the physical sciences. Its applications span a multitude of domains such as particle physics, cosmology, quantum many-body physics, quantum computing, and chemical and material physics. The collaboration between ML and physical sciences has led to conceptual advancements in ML, inspired by physical insights, and vice versa. This synergy has not only facilitated a deeper understanding of physical phenomena but also accelerated advancements in computational techniques, showcasing the multifaceted benefits of ML in scientific exploration (Carleo et al., 2019).
Machine Learning in Nuclear Data Evaluation
Machine learning has also proven to be instrumental in the field of nuclear data evaluation. The predictive capabilities of ML models have been successfully employed to infer neutron-induced reaction cross-sections, demonstrating superior accuracy compared to traditional physics-guided models. This approach mitigates human bias, reduces uncertainties, and enables faster, more reliable decision-making in nuclear data evaluation. The integration of ML in this domain exemplifies its potential to refine and expedite complex evaluative tasks (Vicente-Valdez et al., 2021).
Machine Learning in Seismology
In seismology, ML has emerged as a transformative force, enabling the identification of unseen signals and patterns, and enhancing the understanding of seismic phenomena. Applications range from earthquake detection and early warning systems to ground-motion prediction and seismic tomography. The adoption of ML in seismology underscores its capability to amalgamate data-driven insights with traditional physical modeling, paving the way for comprehensive seismic analysis and prediction (Kong et al., 2018).
Machine Learning in Cardiac Electrophysiology and Arrhythmias
ML's application extends to the field of cardiac electrophysiology and arrhythmias, where it offers novel pathways for understanding arrhythmia mechanisms and improving the techniques for mapping cardiac electrical function. The versatility of ML in analyzing large datasets and identifying key features is particularly beneficial in this domain, facilitating the development of predictive models and aiding in decision-making for arrhythmia management (Trayanova et al., 2021).
特性
分子式 |
C19H21NO4S |
---|---|
分子量 |
359.44 |
同義語 |
(E)-4-Methyl-5-(((phenylsulfonyl)oxy)imino)-[1,1/'-bi(cyclohexane)]-3,6-dien-2-one |
製品の起源 |
United States |
試験管内研究製品の免責事項と情報
BenchChemで提示されるすべての記事および製品情報は、情報提供を目的としています。BenchChemで購入可能な製品は、生体外研究のために特別に設計されています。生体外研究は、ラテン語の "in glass" に由来し、生物体の外で行われる実験を指します。これらの製品は医薬品または薬として分類されておらず、FDAから任何の医療状態、病気、または疾患の予防、治療、または治癒のために承認されていません。これらの製品を人間または動物に体内に導入する形態は、法律により厳格に禁止されています。これらのガイドラインに従うことは、研究と実験において法的および倫理的な基準の遵守を確実にするために重要です。