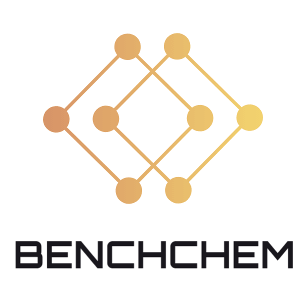
ML 179
カタログ番号 B1150228
分子量: 422.44
InChIキー:
注意: 研究専用です。人間または獣医用ではありません。
- 専門家チームからの見積もりを受け取るには、QUICK INQUIRYをクリックしてください。
- 品質商品を競争力のある価格で提供し、研究に集中できます。
説明
Selective liver receptor homolog 1 (LRH1, NR5A2) inverse agonist (IC50 = 320 nM and maximum efficacy of 64% repression). Exhibits >31-fold selectivity over SF1.
科学的研究の応用
Machine Learning in the Physical Sciences
- Overview : Machine learning has been applied across various domains in physics, including particle physics, cosmology, quantum computing, and chemical and material physics. ML has also spurred research and development in computing architectures to accelerate ML applications.
- Key Insights : ML's integration into physics has led to new conceptual developments in ML, driven by physical insights, and has significantly enhanced our understanding in areas like quantum many-body physics and seismic tomography.
- References : (Carleo et al., 2019).
Machine Learning in Ophthalmology
- Overview : ML, including deep learning, has been applied extensively in ophthalmology, especially in diagnosing conditions like diabetic retinopathy and glaucoma using optical coherence tomography images.
- Key Insights : This area of research is rapidly growing, with the USA, England, and China being the leading countries in this field. The integration of AI in ophthalmology signifies a major shift in diagnosing and managing eye diseases.
- References : (Wang et al., 2021).
Machine Learning in Seismology
- Overview : ML techniques are increasingly being used in seismology for earthquake detection, early warning systems, and understanding seismic tomography.
- Key Insights : ML helps in identifying unseen signals and patterns in seismic data, potentially improving our ability to predict and respond to seismic events.
- References : (Kong et al., 2018).
Machine Learning in Materials Science
- Overview : ML methods are transforming materials science, particularly in the design and development of metal-organic frameworks (MOFs).
- Key Insights : ML helps predict material properties and design new structures, potentially revolutionizing materials research and development.
- References : (Chong et al., 2020).
Machine Learning in Cancer Research
- Overview : ML methods have been applied in cancer research for prognosis and prediction, utilizing techniques like Artificial Neural Networks and Support Vector Machines.
- Key Insights : ML tools have been instrumental in modeling cancer progression and treatment, enhancing our understanding and management of various cancer types.
- References : (Kourou et al., 2014).
Machine Learning in Environmental Science
- Overview : ML methods are increasingly being used to understand and predict environmental pollution, with a significant focus on air pollution.
- Key Insights : ML can revolutionize environmental science, but challenges remain in terms of model interpretability and data sharing.
- References : (Liu et al., 2022).
特性
分子式 |
C21H25F3N4O2 |
---|---|
分子量 |
422.44 |
同義語 |
3-Cyclohexyl-6-[4-[3-(trifluoromethyl)phenyl]piperazin-1-yl]-1H-pyrimidine-2,4-dione |
製品の起源 |
United States |
試験管内研究製品の免責事項と情報
BenchChemで提示されるすべての記事および製品情報は、情報提供を目的としています。BenchChemで購入可能な製品は、生体外研究のために特別に設計されています。生体外研究は、ラテン語の "in glass" に由来し、生物体の外で行われる実験を指します。これらの製品は医薬品または薬として分類されておらず、FDAから任何の医療状態、病気、または疾患の予防、治療、または治癒のために承認されていません。これらの製品を人間または動物に体内に導入する形態は、法律により厳格に禁止されています。これらのガイドラインに従うことは、研究と実験において法的および倫理的な基準の遵守を確実にするために重要です。
迅速なお問い合わせ
カテゴリー