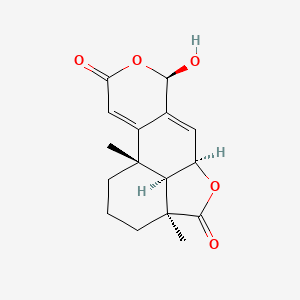
Yukonin
- Cliquez sur DEMANDE RAPIDE pour recevoir un devis de notre équipe d'experts.
- Avec des produits de qualité à un prix COMPÉTITIF, vous pouvez vous concentrer davantage sur votre recherche.
Vue d'ensemble
Description
Yukonin is a natural product found in Clarireedia homoeocarpa and Phialophora with data available.
Applications De Recherche Scientifique
Vegetation Dynamics in Yukon Ecosystems
The dynamic ecosystems of Yukon are undergoing significant shifts due to climate change, impacting vegetation communities, carbon and nutrient cycling, animal habitats, biodiversity, and other ecosystem processes. Recent findings indicate these changes are substantial, predicting shifts in vegetation communities across multiple ecological units or bioclimate zones. Despite the presence of various vegetation communities in Yukon and the paleoecological evidence of past significant changes, there is a lack of comprehensive synthesis, making predictions about future vegetation assemblages, their abundance, and influence challenging. Identifying critical research gaps for each vegetation community and disturbance type is crucial to understand the responses of Yukon bioclimate zones and vegetation communities to future warming and disturbances (Reid, Reid, & Brown, 2022).
Data-Driven Approaches in Diabetes Research
The advancements in biotechnology and health sciences have led to a surge in data production, such as high throughput genetic data and large Electronic Health Records (EHRs). Machine learning and data mining methods have become increasingly indispensable in transforming this information into valuable knowledge, especially in diabetes research. Diabetes Mellitus (DM) is a metabolic disorder exerting significant pressure on human health worldwide. The extensive research in various aspects of diabetes has led to the generation of substantial data. Machine learning, data mining techniques, and tools have been employed in diabetes research, particularly in prediction and diagnosis, diabetic complications, genetic background and environment, and health care and management. These applications project the usefulness of extracting valuable knowledge, leading to new hypotheses, deeper understanding, and further investigation in DM (Kavakiotis et al., 2017).
Application of Scientific Method in Small Catchment Studies
Applying the scientific method iteratively in catchment science, which includes hillslope hydrology and biogeochemistry, can significantly refine our understanding of the processes controlling these phenomena. However, the scale discrepancy between controlled experiments and the phenomena of interest, along with the underutilization of computer simulation models as hypothesis-testing tools, has hindered this progress. The Panola Mountain Research Watershed serves as an example, demonstrating how the iterative application of a simple mixing model can enhance understanding and discern general principles applicable to other studies. This approach underscores the necessity for an explicitly stated conceptual model of the catchment, objective measures of its applicability, and a clear linkage between the observation scale and the prediction scale (Hooper, 2001).
Public Participation in Scientific Research
Public participation in scientific research (PPSR) occurs in diverse contexts, particularly in conservation and natural resource management, where it can significantly affect project design and outcomes. This participation is crucial in addressing complex social-ecological questions. Different models of PPSR show that project outcomes are influenced by the degree of public participation in the research process and the quality of this participation during project design. A framework illustrating the relationships between the quality of participation and outcomes may assist in deliberate design efforts of PPSR initiatives, enhancing outcomes for scientific research, individual participants, and social-ecological systems (Shirk et al., 2012).
Propriétés
Nom du produit |
Yukonin |
---|---|
Formule moléculaire |
C16H18O5 |
Poids moléculaire |
290.31 g/mol |
Nom IUPAC |
(1S,6R,9R,12S,16R)-6-hydroxy-1,12-dimethyl-5,10-dioxatetracyclo[7.6.1.02,7.012,16]hexadeca-2,7-diene-4,11-dione |
InChI |
InChI=1S/C16H18O5/c1-15-4-3-5-16(2)12(15)10(20-14(16)19)6-8-9(15)7-11(17)21-13(8)18/h6-7,10,12-13,18H,3-5H2,1-2H3/t10-,12-,13-,15-,16+/m1/s1 |
Clé InChI |
PTNNCNNWQDJWHA-MQWZGVGHSA-N |
SMILES isomérique |
C[C@]12CCC[C@]3([C@@H]1[C@@H](C=C4C2=CC(=O)O[C@H]4O)OC3=O)C |
SMILES |
CC12CCCC3(C1C(C=C4C2=CC(=O)OC4O)OC3=O)C |
SMILES canonique |
CC12CCCC3(C1C(C=C4C2=CC(=O)OC4O)OC3=O)C |
Synonymes |
yukonin |
Origine du produit |
United States |
Retrosynthesis Analysis
AI-Powered Synthesis Planning: Our tool employs the Template_relevance Pistachio, Template_relevance Bkms_metabolic, Template_relevance Pistachio_ringbreaker, Template_relevance Reaxys, Template_relevance Reaxys_biocatalysis model, leveraging a vast database of chemical reactions to predict feasible synthetic routes.
One-Step Synthesis Focus: Specifically designed for one-step synthesis, it provides concise and direct routes for your target compounds, streamlining the synthesis process.
Accurate Predictions: Utilizing the extensive PISTACHIO, BKMS_METABOLIC, PISTACHIO_RINGBREAKER, REAXYS, REAXYS_BIOCATALYSIS database, our tool offers high-accuracy predictions, reflecting the latest in chemical research and data.
Strategy Settings
Precursor scoring | Relevance Heuristic |
---|---|
Min. plausibility | 0.01 |
Model | Template_relevance |
Template Set | Pistachio/Bkms_metabolic/Pistachio_ringbreaker/Reaxys/Reaxys_biocatalysis |
Top-N result to add to graph | 6 |
Feasible Synthetic Routes
Avertissement et informations sur les produits de recherche in vitro
Veuillez noter que tous les articles et informations sur les produits présentés sur BenchChem sont destinés uniquement à des fins informatives. Les produits disponibles à l'achat sur BenchChem sont spécifiquement conçus pour des études in vitro, qui sont réalisées en dehors des organismes vivants. Les études in vitro, dérivées du terme latin "in verre", impliquent des expériences réalisées dans des environnements de laboratoire contrôlés à l'aide de cellules ou de tissus. Il est important de noter que ces produits ne sont pas classés comme médicaments et n'ont pas reçu l'approbation de la FDA pour la prévention, le traitement ou la guérison de toute condition médicale, affection ou maladie. Nous devons souligner que toute forme d'introduction corporelle de ces produits chez les humains ou les animaux est strictement interdite par la loi. Il est essentiel de respecter ces directives pour assurer la conformité aux normes légales et éthiques en matière de recherche et d'expérimentation.