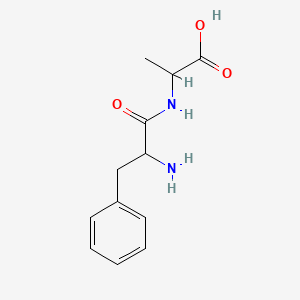
Phenylalanyl-alanine
Descripción general
Descripción
Phenylalanyl-alanine, also known as F-a dipeptide or phe-ala, belongs to the class of organic compounds known as dipeptides. These are organic compounds containing a sequence of exactly two alpha-amino acids joined by a peptide bond. Phenylalanyl-alanine is slightly soluble (in water) and a weakly acidic compound (based on its pKa). Phenylalanyl-alanine has been primarily detected in feces.
Aplicaciones Científicas De Investigación
Probe for Peptide Transporter 1
L-Phenylalanyl-Ψ[CS-N]-l-alanine (Phe-Ψ-Ala), a thiourea dipeptide, has been evaluated as a probe for peptide transporter 1 (PEPT1). It exhibits high affinity and metabolic stability, making it a useful non-radioactive probe for studying PEPT1, potentially aiding in predicting drug-drug interactions mediated by PEPT1 both in vitro and in vivo (Arakawa et al., 2014).
Molecular Arrangement in Crystal Structure
The crystal structure of L-phenylalanyl-L-alanine dihydrate reveals a new type of molecular arrangement for dipeptides. In this structure, L-Phe and L-Ala side chains aggregate into hydrophobic columns within a hydrogen-bond network, contributing to our understanding of dipeptide structural behavior (Görbitz, 2001).
Genetic Code Exploration
Research involving a mutant yeast phenylalanine transfer RNA with a modified anticodon demonstrates the feasibility of altering the genetic code in Escherichia coli. This alteration enabled efficient replacement of phenylalanine by L-3-(2-naphthyl)alanine at specific codons, providing insights into genetic code manipulation (Kwon, Kirshenbaum, & Tirrell, 2003).
Aggregation and Toxicity of Phenylalanine
Studies on phenylalanine oligomers and fibrils, related to Phenylketonuria, reveal their assembly mechanism and cytotoxic properties. This research enhances our understanding of the molecular basis of Phenylketonuria and the potential for targeted therapeutic strategies (Do, Kincannon, & Bowers, 2015).
Antimicrobial Applications
Research on Phenylalanyl tRNA synthetase (PheRS) substrate mimics has led to the design, synthesis, and evaluation of novel compounds for antimicrobial purposes. This highlights the potential of PheRS as a target for developing new antimicrobial agents (Noureldin et al., 2022).
Interaction with Micelles
Research on the interaction of alanyl-phenylalanyl-alanine with cesium perfluorooctanoate micelles contributes to understanding peptide-micelle interactions, a crucial aspect in studying peptide behavior in various environments (Pizzanelli, Forte, Monti, & Schweitzer‐Stenner, 2008).
Propiedades
IUPAC Name |
2-[(2-amino-3-phenylpropanoyl)amino]propanoic acid | |
---|---|---|
Details | Computed by Lexichem TK 2.7.0 (PubChem release 2021.05.07) | |
Source | PubChem | |
URL | https://pubchem.ncbi.nlm.nih.gov | |
Description | Data deposited in or computed by PubChem | |
InChI |
InChI=1S/C12H16N2O3/c1-8(12(16)17)14-11(15)10(13)7-9-5-3-2-4-6-9/h2-6,8,10H,7,13H2,1H3,(H,14,15)(H,16,17) | |
Details | Computed by InChI 1.0.6 (PubChem release 2021.05.07) | |
Source | PubChem | |
URL | https://pubchem.ncbi.nlm.nih.gov | |
Description | Data deposited in or computed by PubChem | |
InChI Key |
MIDZLCFIAINOQN-UHFFFAOYSA-N | |
Details | Computed by InChI 1.0.6 (PubChem release 2021.05.07) | |
Source | PubChem | |
URL | https://pubchem.ncbi.nlm.nih.gov | |
Description | Data deposited in or computed by PubChem | |
Canonical SMILES |
CC(C(=O)O)NC(=O)C(CC1=CC=CC=C1)N | |
Details | Computed by OEChem 2.3.0 (PubChem release 2021.05.07) | |
Source | PubChem | |
URL | https://pubchem.ncbi.nlm.nih.gov | |
Description | Data deposited in or computed by PubChem | |
Molecular Formula |
C12H16N2O3 | |
Details | Computed by PubChem 2.1 (PubChem release 2021.05.07) | |
Source | PubChem | |
URL | https://pubchem.ncbi.nlm.nih.gov | |
Description | Data deposited in or computed by PubChem | |
DSSTOX Substance ID |
DTXSID301001642 | |
Record name | N-(2-Amino-1-hydroxy-3-phenylpropylidene)alanine | |
Source | EPA DSSTox | |
URL | https://comptox.epa.gov/dashboard/DTXSID301001642 | |
Description | DSSTox provides a high quality public chemistry resource for supporting improved predictive toxicology. | |
Molecular Weight |
236.27 g/mol | |
Details | Computed by PubChem 2.1 (PubChem release 2021.05.07) | |
Source | PubChem | |
URL | https://pubchem.ncbi.nlm.nih.gov | |
Description | Data deposited in or computed by PubChem | |
Product Name |
Phenylalanyl-alanine | |
CAS RN |
80924-58-9 | |
Record name | NSC524461 | |
Source | DTP/NCI | |
URL | https://dtp.cancer.gov/dtpstandard/servlet/dwindex?searchtype=NSC&outputformat=html&searchlist=524461 | |
Description | The NCI Development Therapeutics Program (DTP) provides services and resources to the academic and private-sector research communities worldwide to facilitate the discovery and development of new cancer therapeutic agents. | |
Explanation | Unless otherwise indicated, all text within NCI products is free of copyright and may be reused without our permission. Credit the National Cancer Institute as the source. | |
Record name | N-(2-Amino-1-hydroxy-3-phenylpropylidene)alanine | |
Source | EPA DSSTox | |
URL | https://comptox.epa.gov/dashboard/DTXSID301001642 | |
Description | DSSTox provides a high quality public chemistry resource for supporting improved predictive toxicology. | |
Retrosynthesis Analysis
AI-Powered Synthesis Planning: Our tool employs the Template_relevance Pistachio, Template_relevance Bkms_metabolic, Template_relevance Pistachio_ringbreaker, Template_relevance Reaxys, Template_relevance Reaxys_biocatalysis model, leveraging a vast database of chemical reactions to predict feasible synthetic routes.
One-Step Synthesis Focus: Specifically designed for one-step synthesis, it provides concise and direct routes for your target compounds, streamlining the synthesis process.
Accurate Predictions: Utilizing the extensive PISTACHIO, BKMS_METABOLIC, PISTACHIO_RINGBREAKER, REAXYS, REAXYS_BIOCATALYSIS database, our tool offers high-accuracy predictions, reflecting the latest in chemical research and data.
Strategy Settings
Precursor scoring | Relevance Heuristic |
---|---|
Min. plausibility | 0.01 |
Model | Template_relevance |
Template Set | Pistachio/Bkms_metabolic/Pistachio_ringbreaker/Reaxys/Reaxys_biocatalysis |
Top-N result to add to graph | 6 |
Feasible Synthetic Routes
Descargo de responsabilidad e información sobre productos de investigación in vitro
Tenga en cuenta que todos los artículos e información de productos presentados en BenchChem están destinados únicamente con fines informativos. Los productos disponibles para la compra en BenchChem están diseñados específicamente para estudios in vitro, que se realizan fuera de organismos vivos. Los estudios in vitro, derivados del término latino "in vidrio", involucran experimentos realizados en entornos de laboratorio controlados utilizando células o tejidos. Es importante tener en cuenta que estos productos no se clasifican como medicamentos y no han recibido la aprobación de la FDA para la prevención, tratamiento o cura de ninguna condición médica, dolencia o enfermedad. Debemos enfatizar que cualquier forma de introducción corporal de estos productos en humanos o animales está estrictamente prohibida por ley. Es esencial adherirse a estas pautas para garantizar el cumplimiento de los estándares legales y éticos en la investigación y experimentación.